## 向量点积的几何意义
**向量点积代数定义**
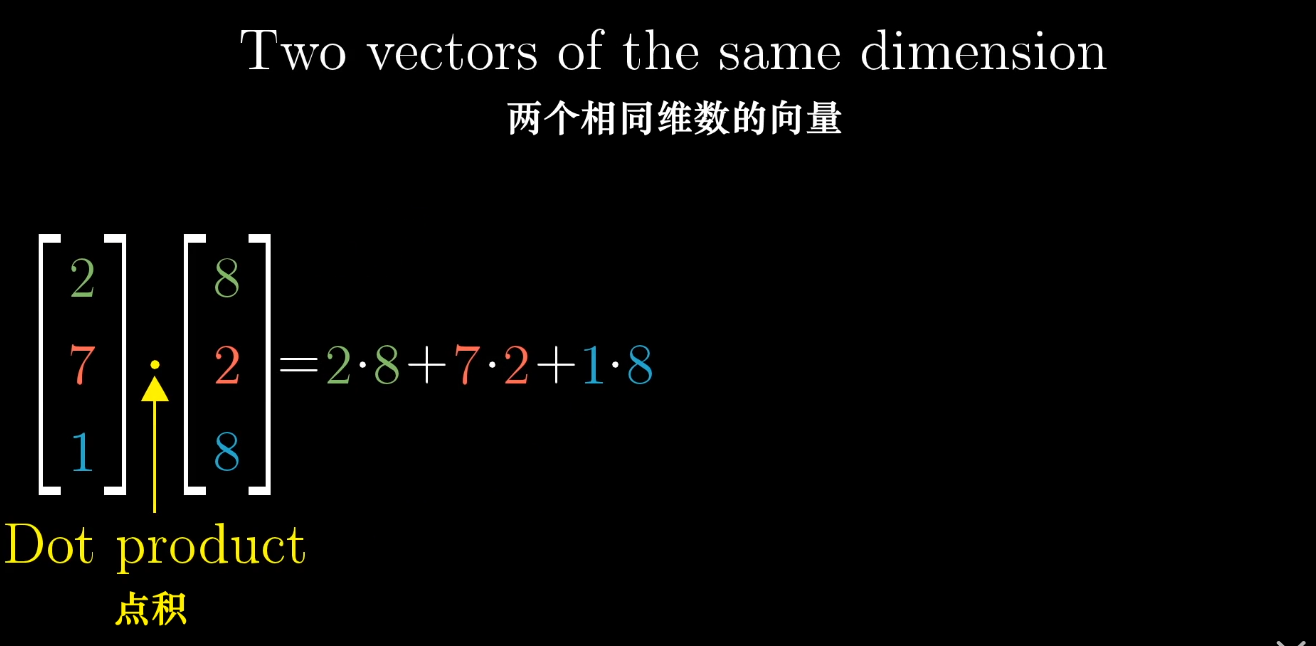
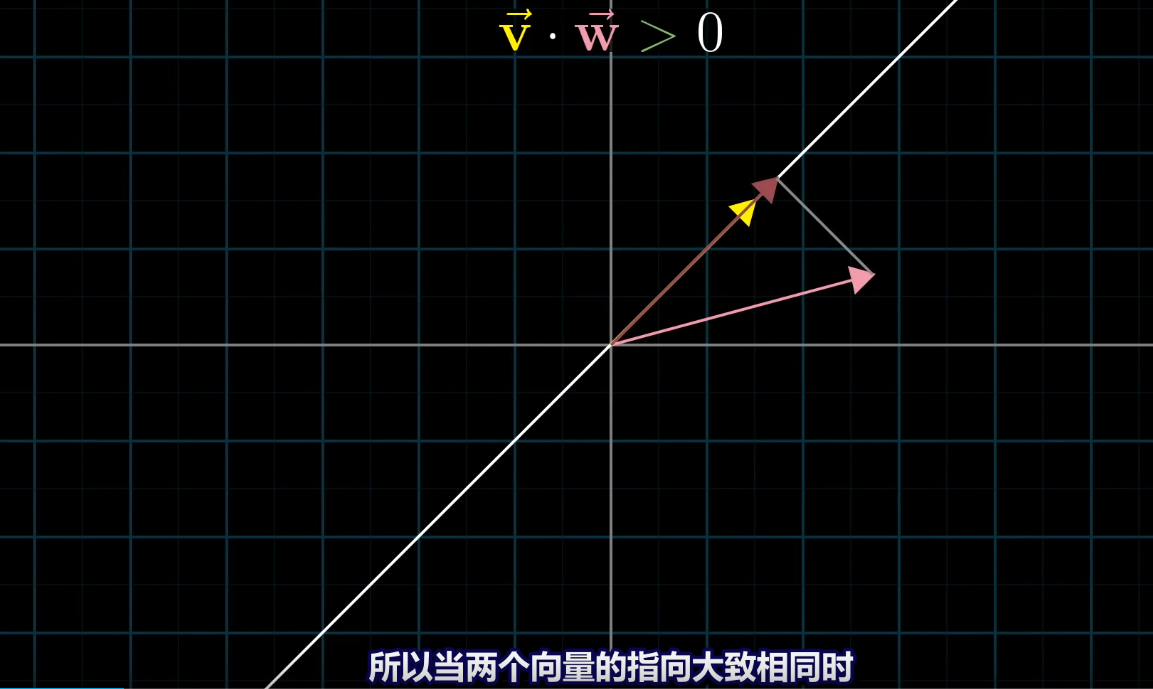
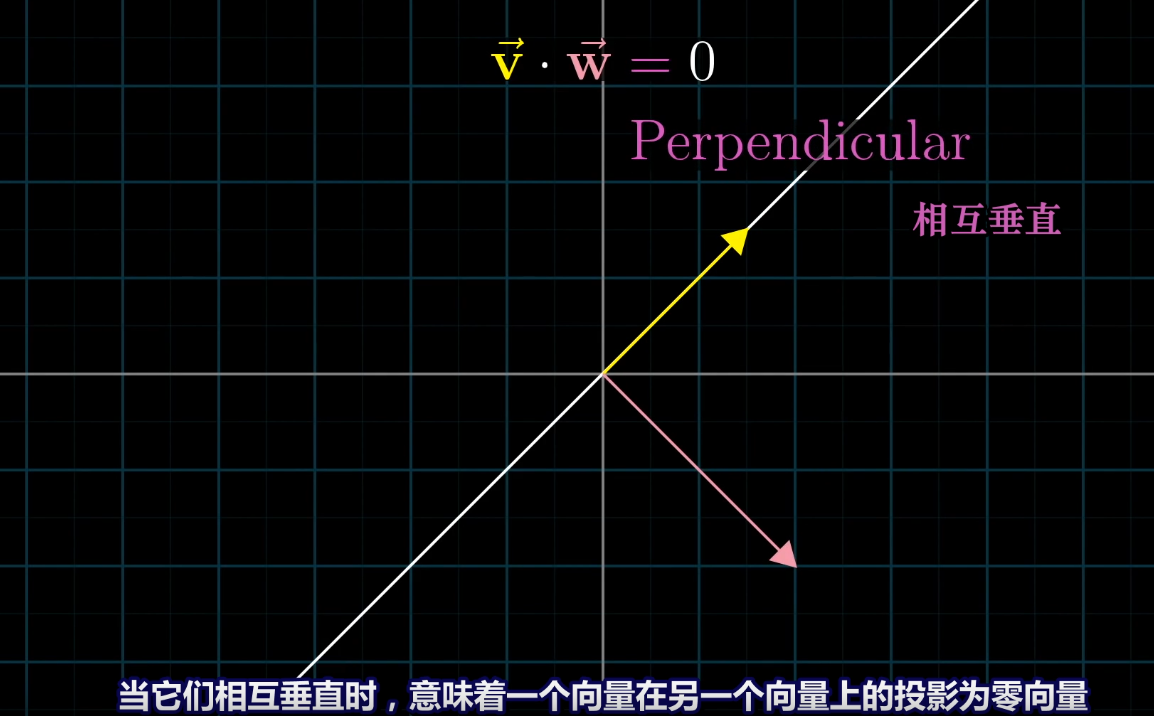
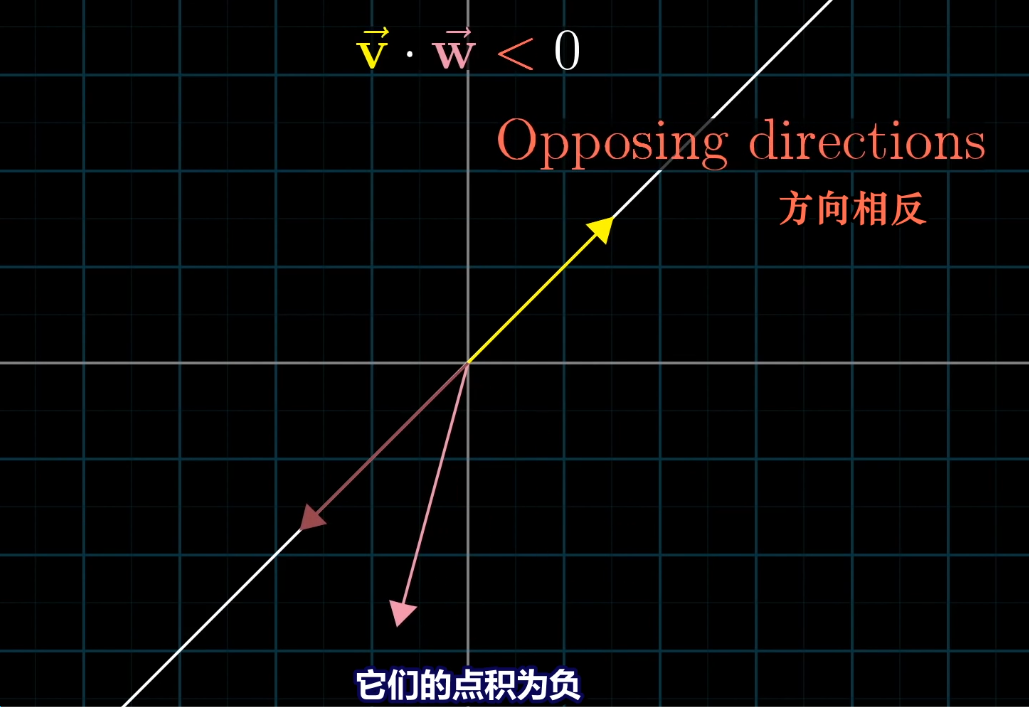
根据对称性,v和w互相投射结果都一样
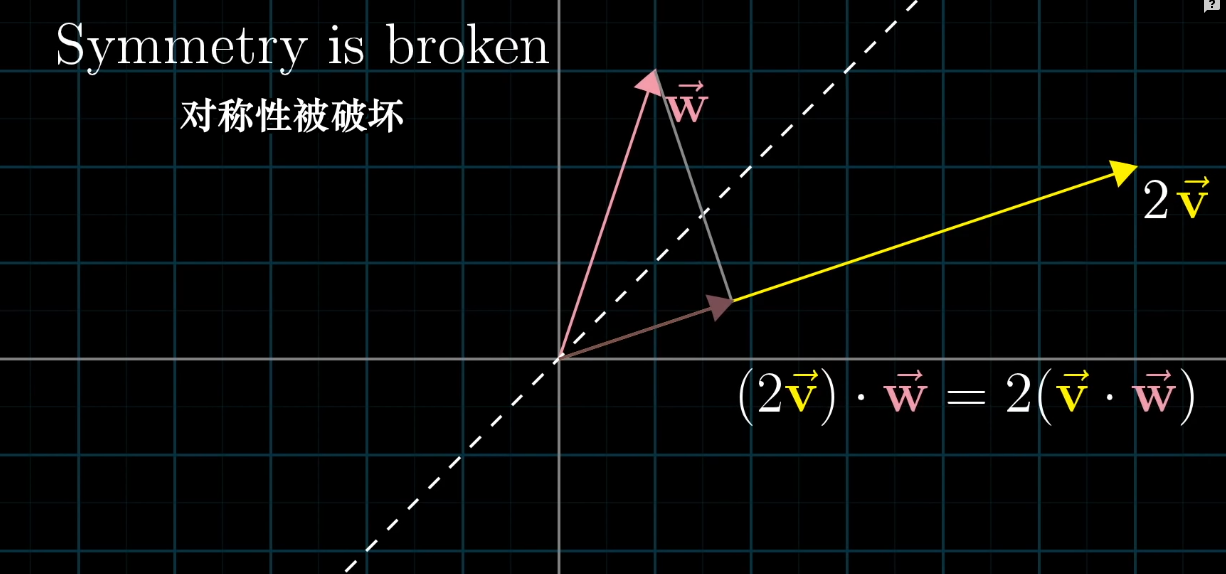
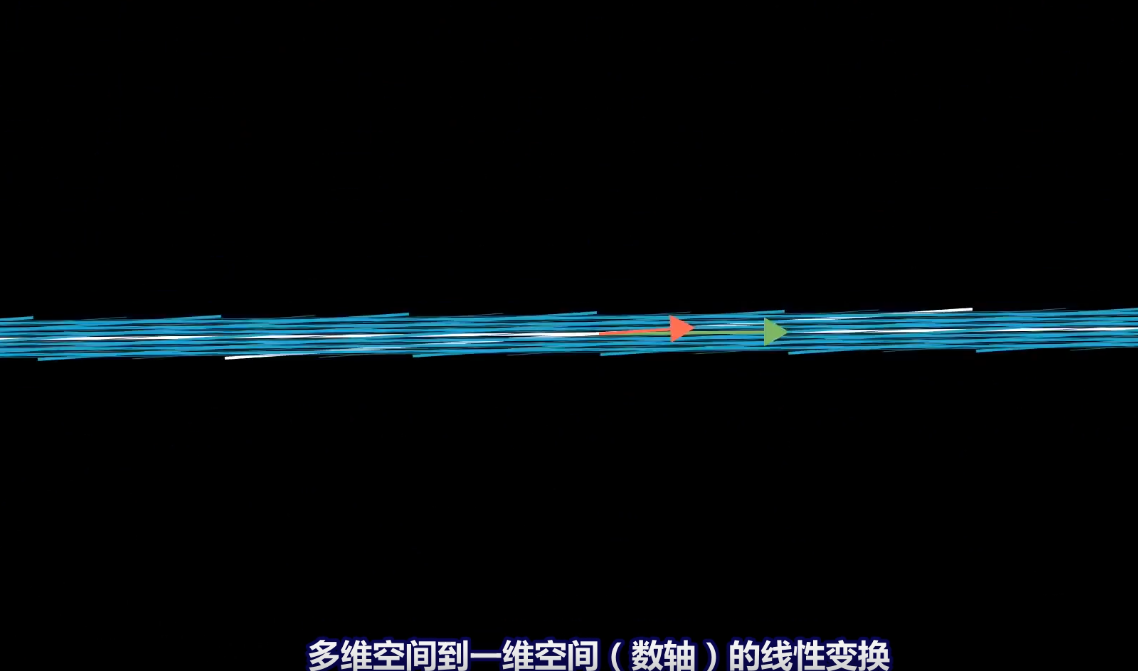
*****
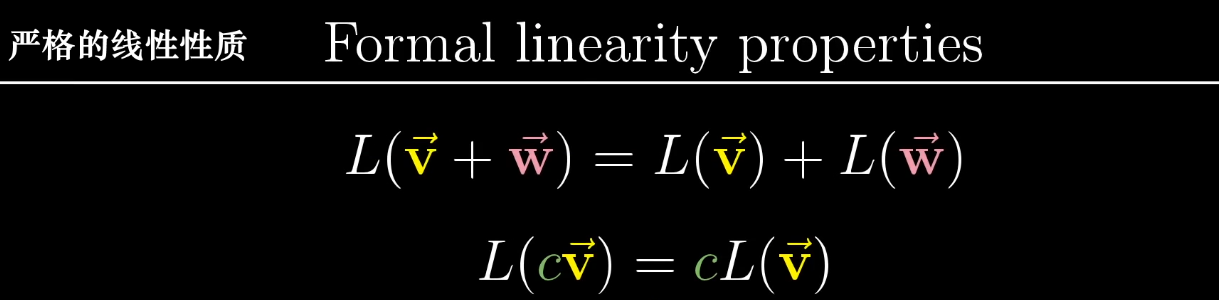
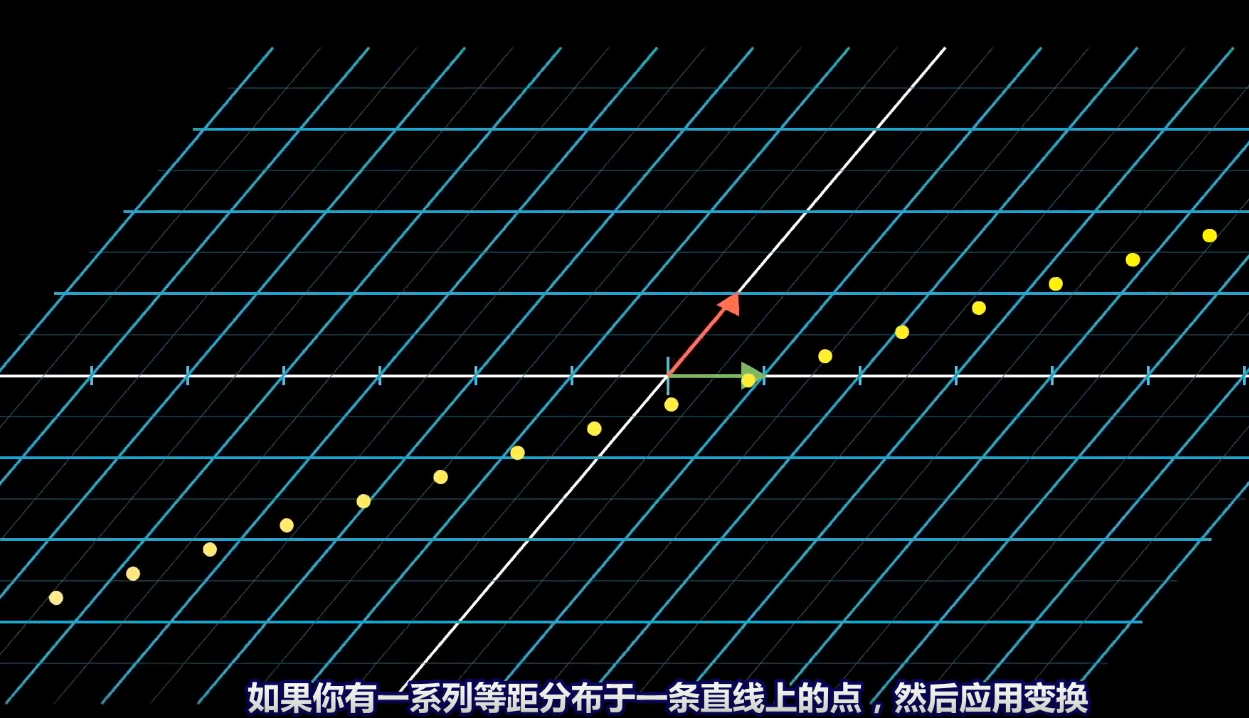
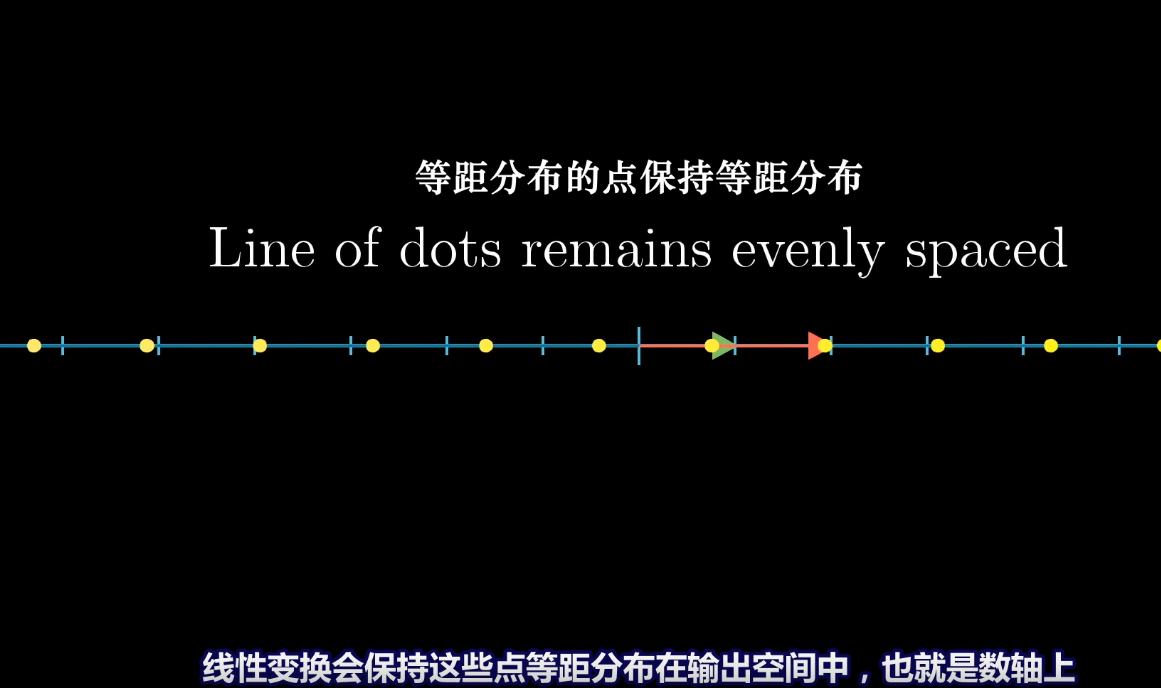
*****
**点积的代数定义**
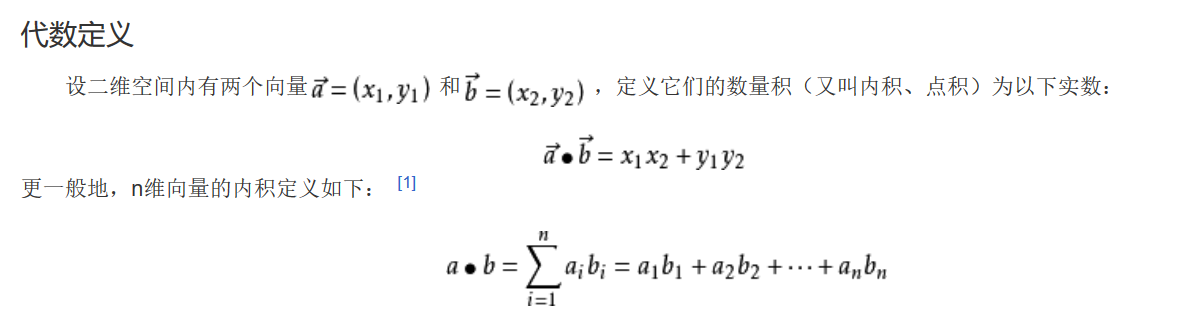
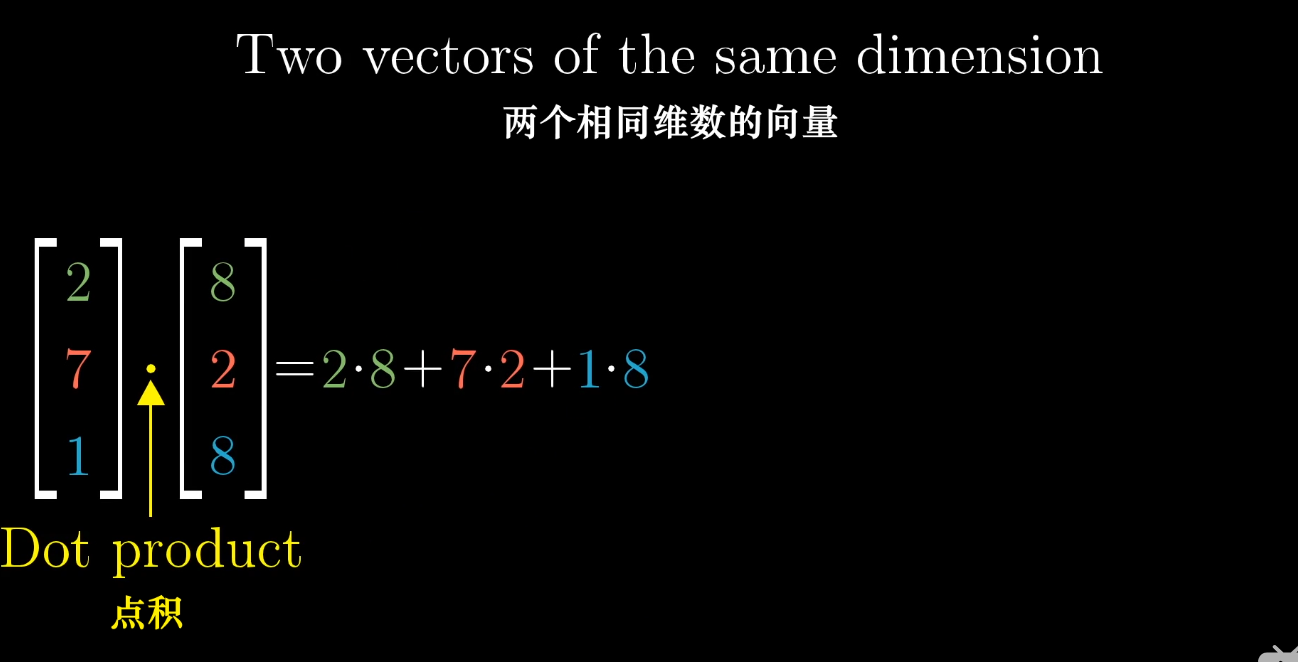
*****
**1*2矩阵的几何意义**
这个矩阵是一个线性变换,表示二维空间的两个基向量i和j通过线性变换,两个基向量落在一个一维数轴上,i和j的坐标对应与x轴重合的数轴的2和1两个点
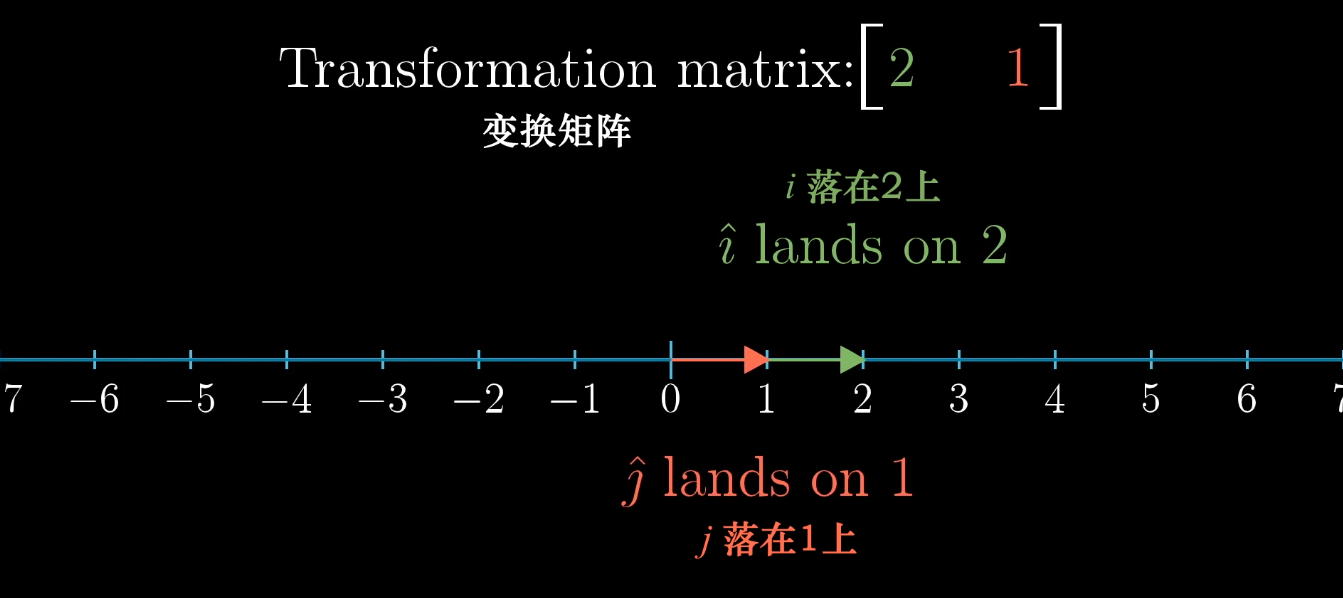
对一个向量向量进行[1 -2]的线性变换,最后向量与1*2矩阵的乘积为2。因为i落在1上,j落在-2上。该向量由4个i和3个j合成,如下图,该向量的x方向分量落在4上,y方向分量落在-6上,4+(-6) = -2
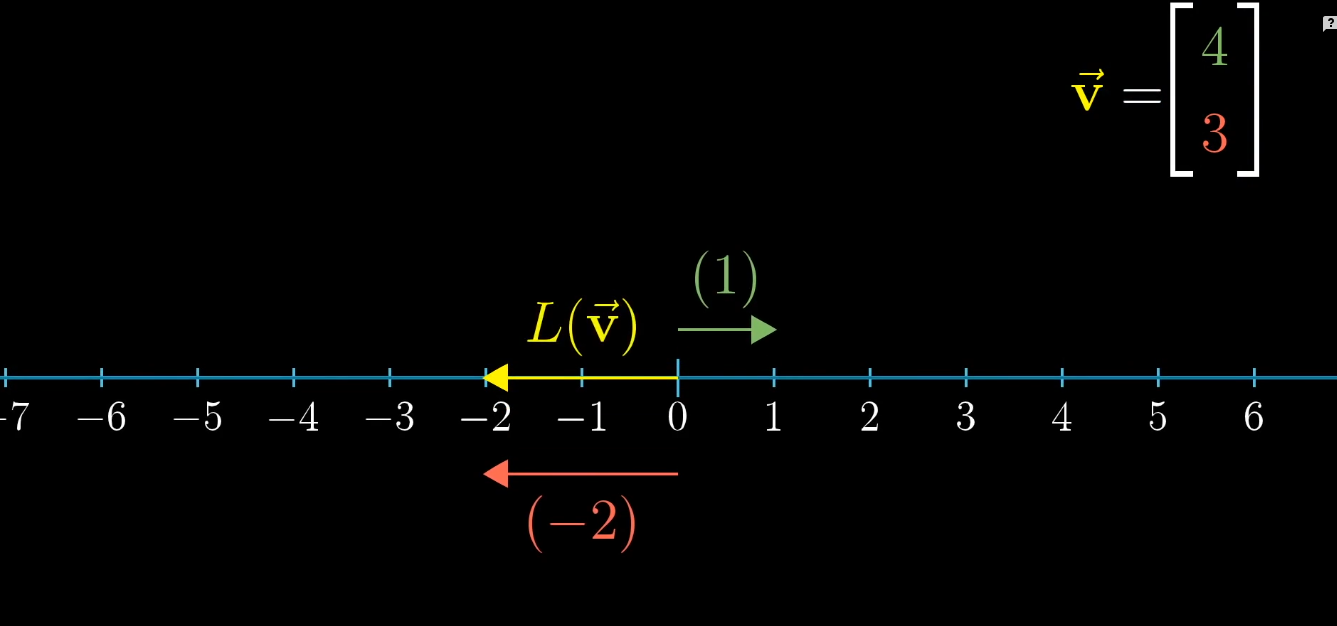
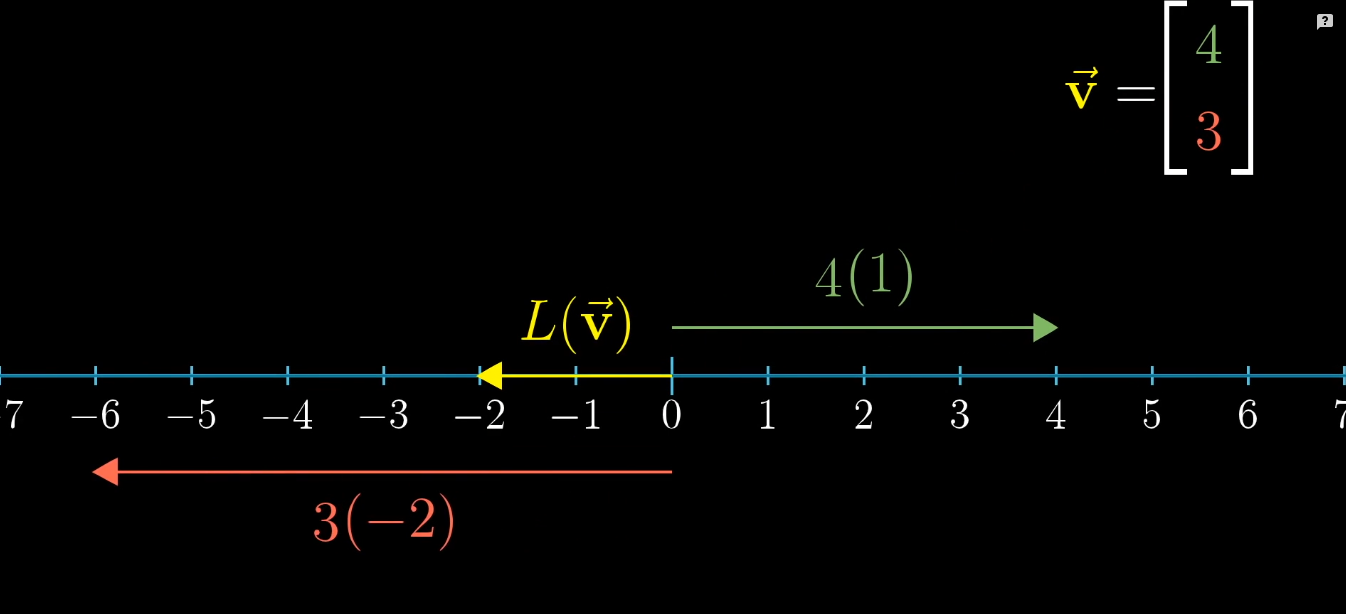
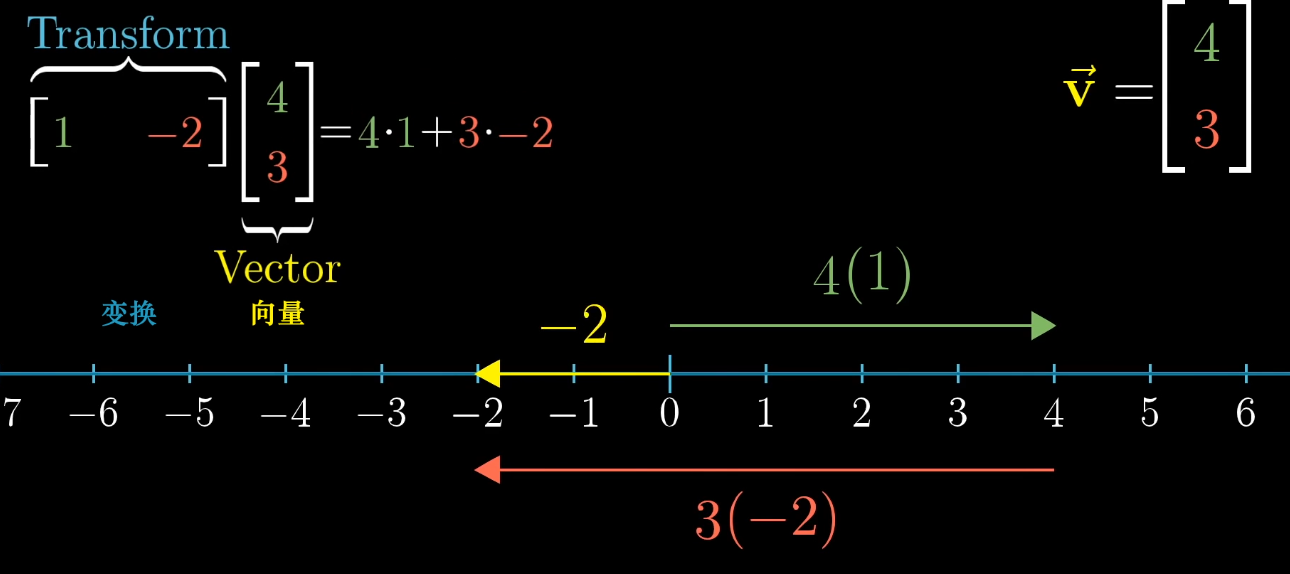
**该变换根据以下线性规则:**
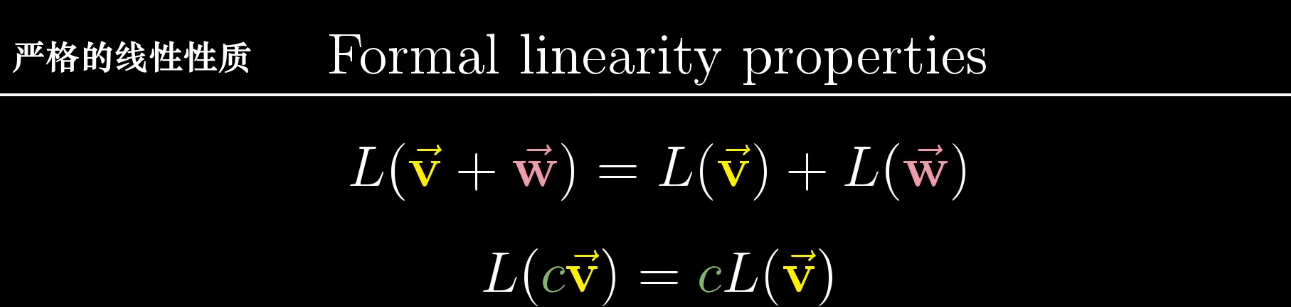
*****
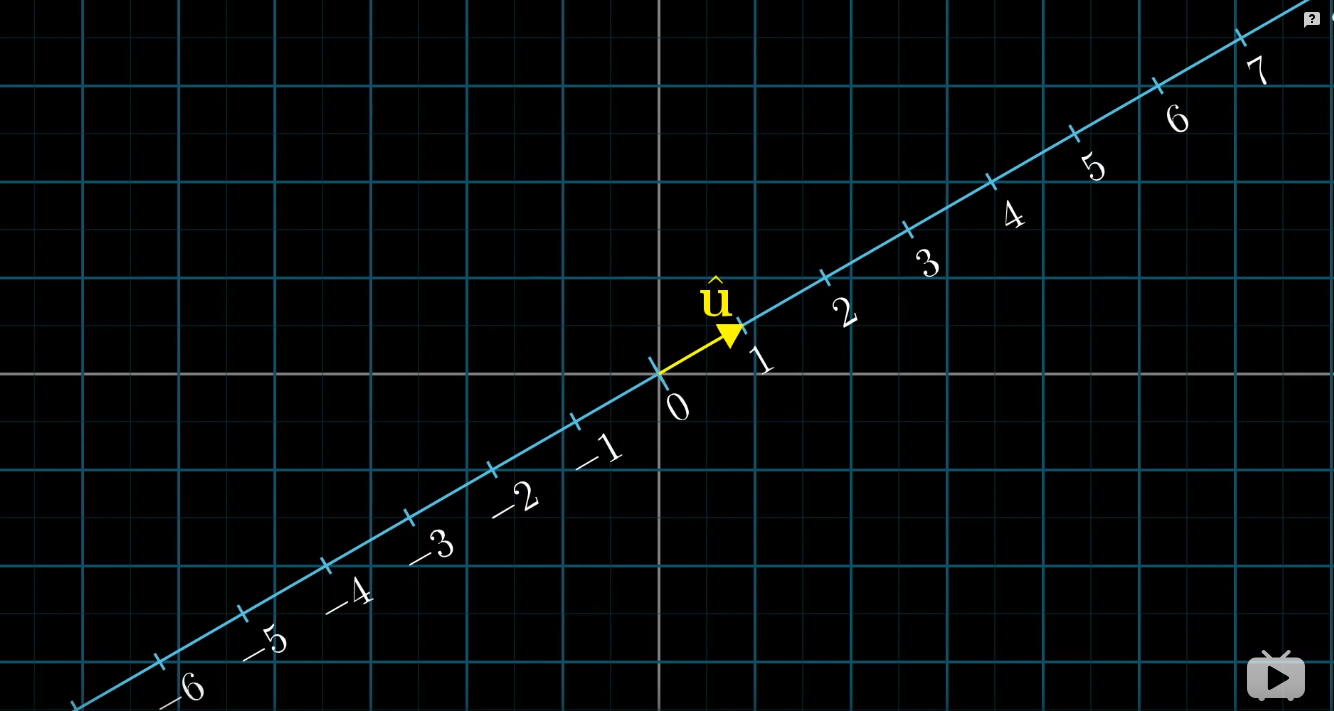
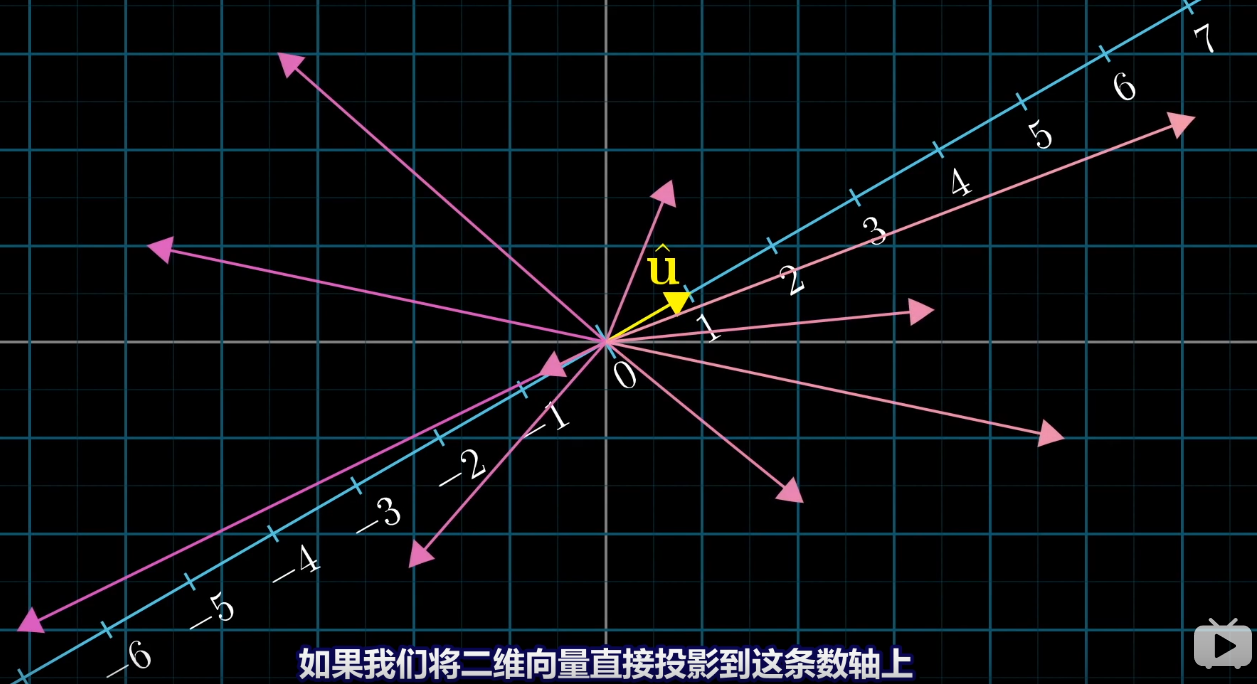
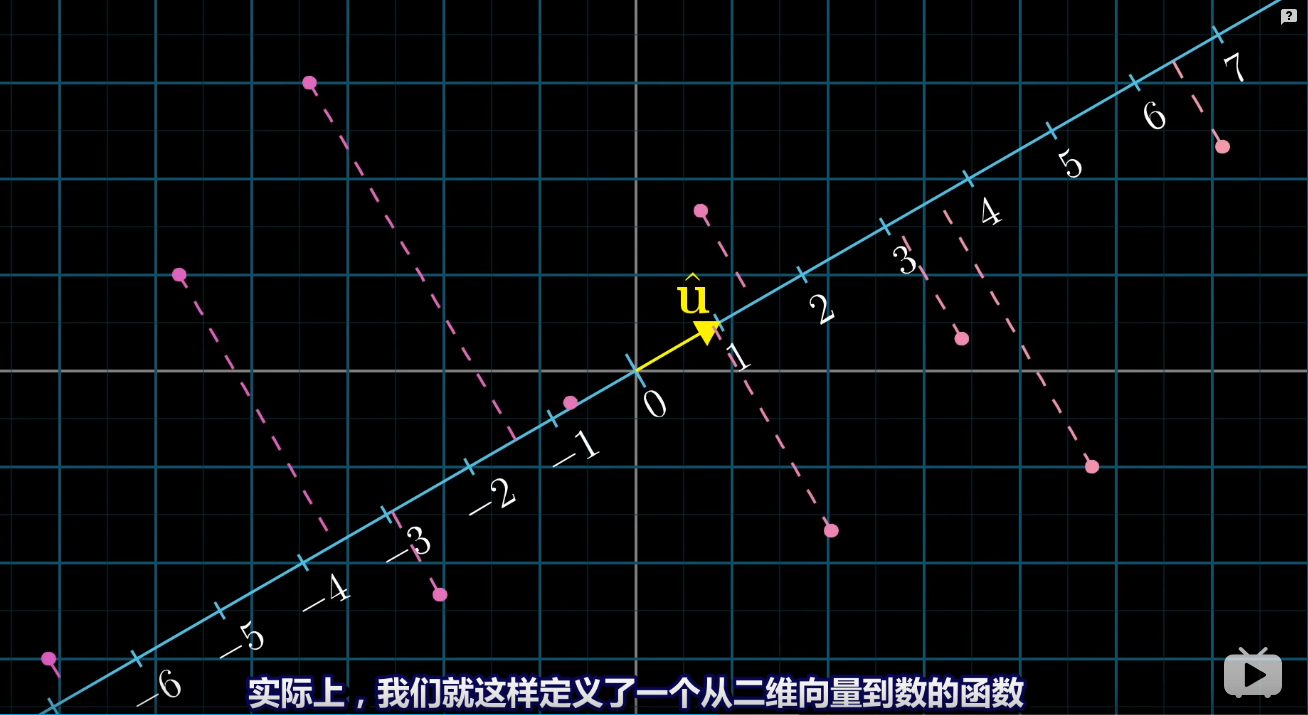
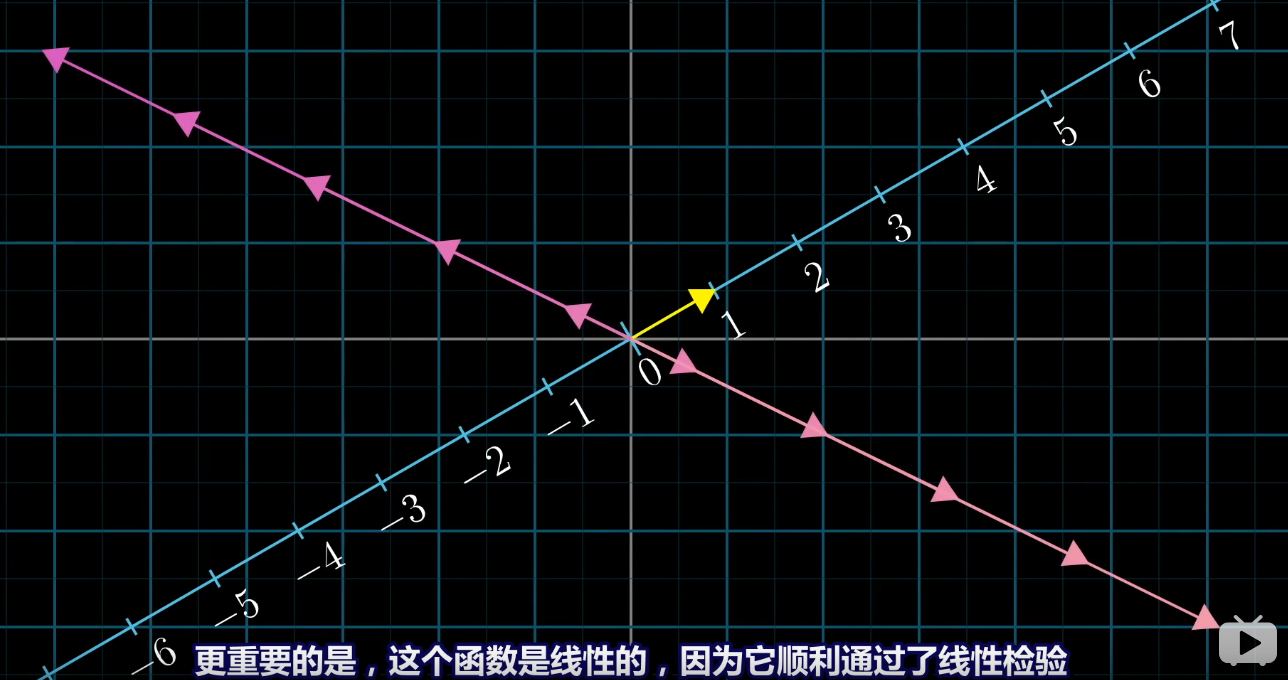
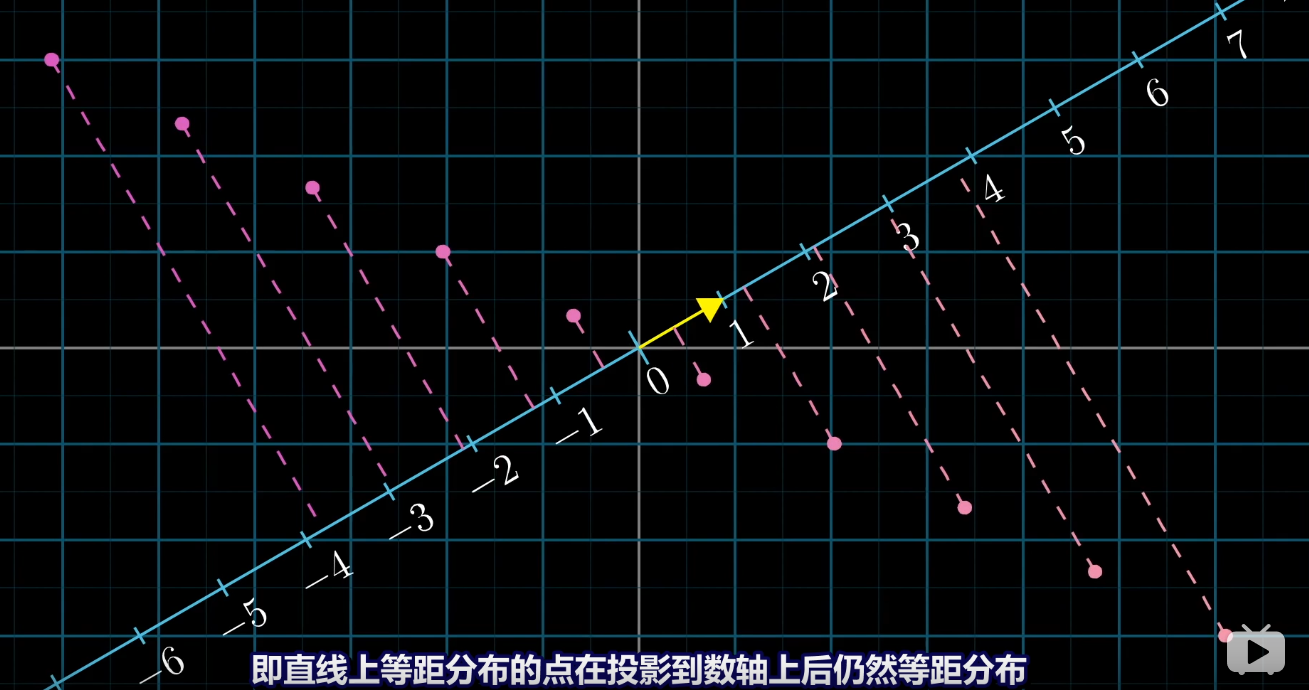
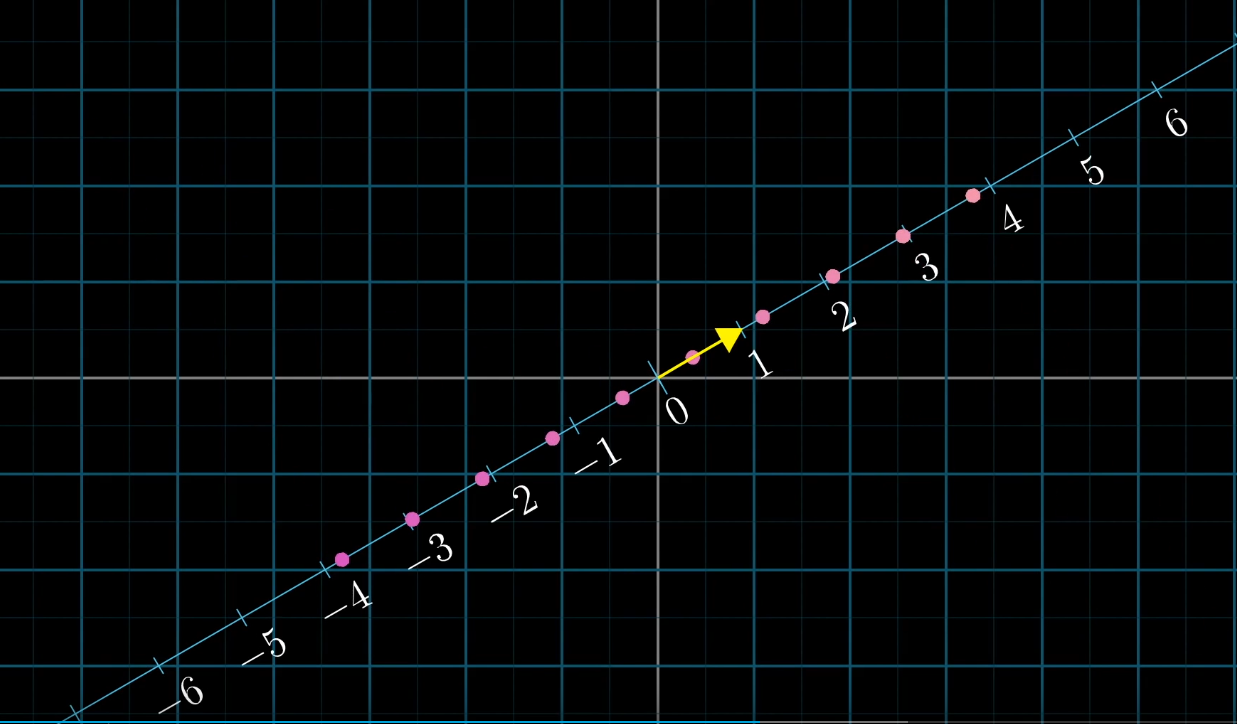
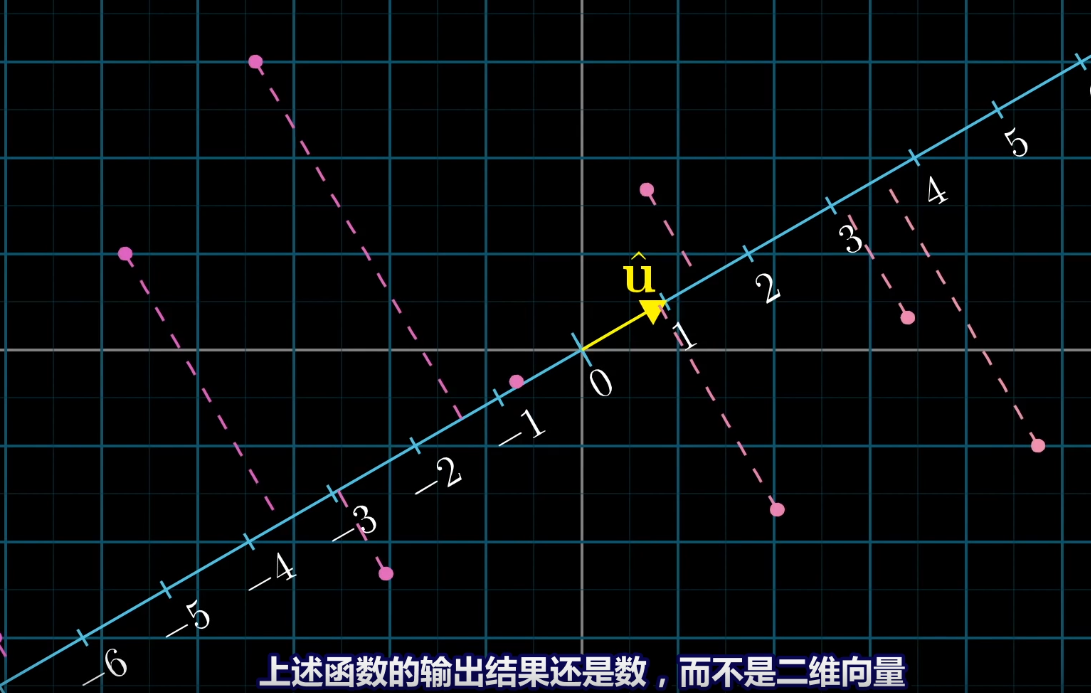
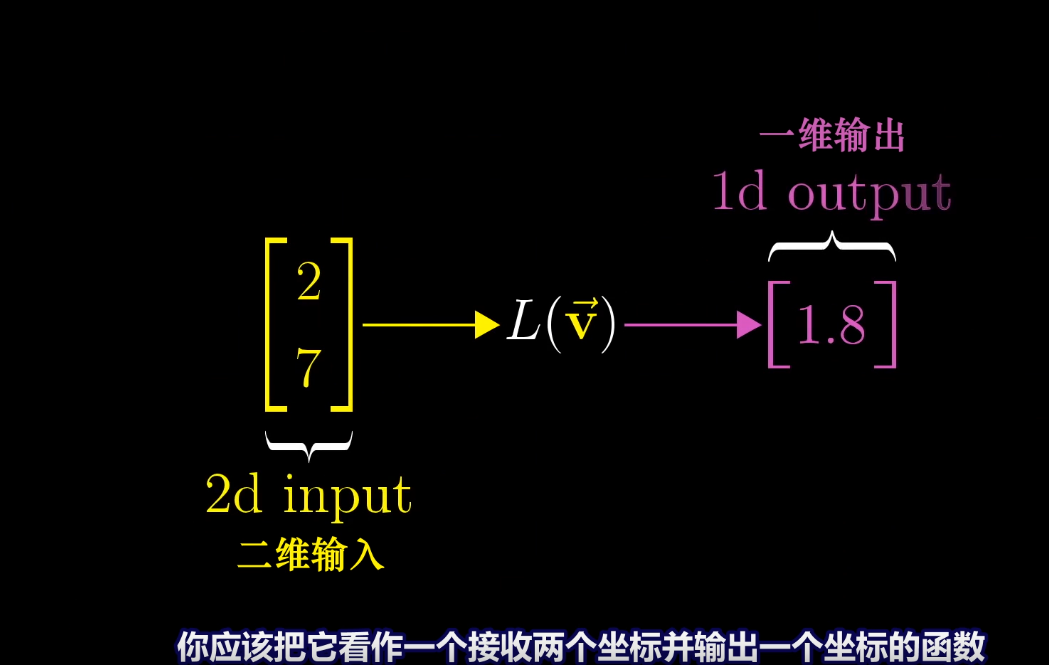
*****
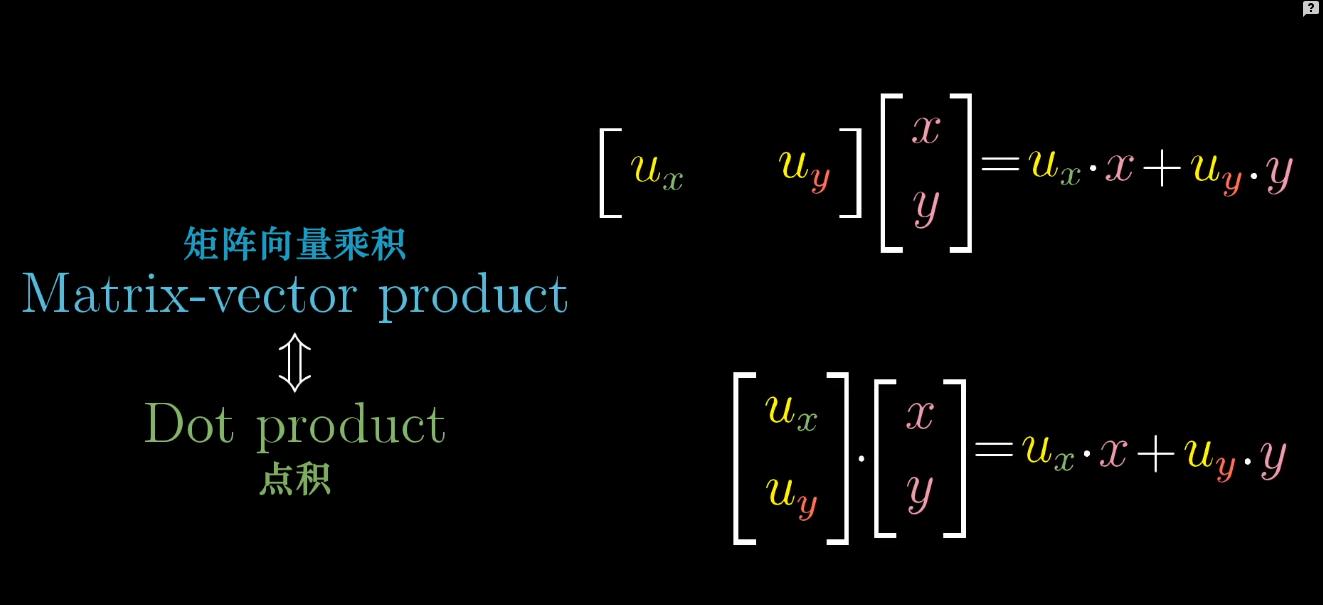
*****
基向量u的坐标为[ux uy] , ux与uy也是基向量i与基向量j投影在基向量上的值(根据作图,对偶性可证)。
i与u的点积=ux*1(基向量u的长度)=ux, j与u的点积=uy*1(基向量u的长度) , ux+uy 等于 * (基向量u),根据下图的原则 :
**1.投影是线性的变换**
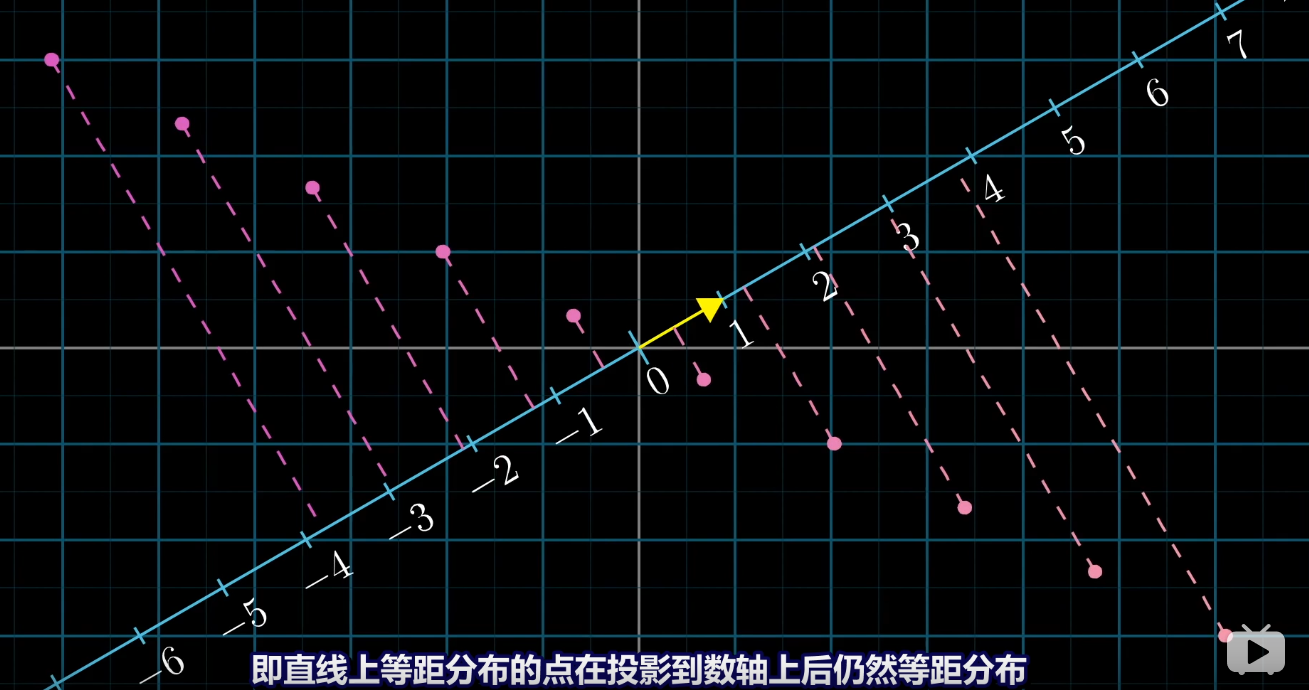
**2. 一个向量的线性变换与它分量变换的和相同**
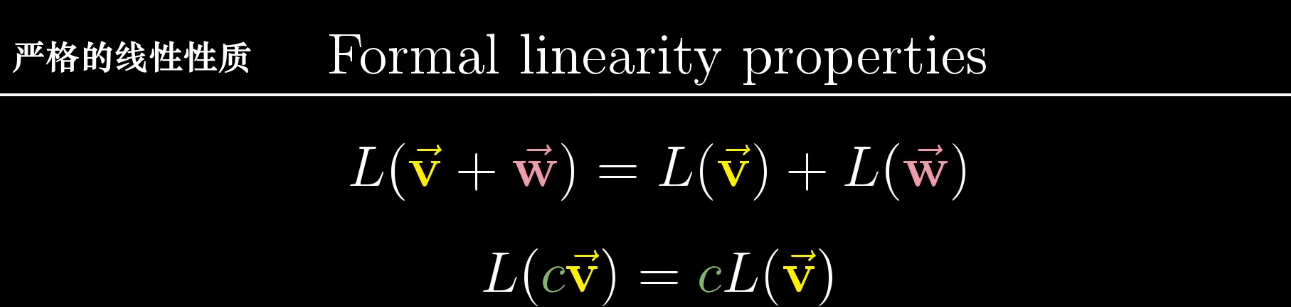
**向量相等,长度一样,方向一样**
*****
任一一向量v 都可以看做n*u(这个向量方向上的基向量),坐标为(n*ux,n*uy)。ux和uy为二维基向量i和j的投影。 [n*ux , n*ny] *  , 那么向量  在 向量v上投影后的数值为=》a*ux*n+b*uy*n,所以
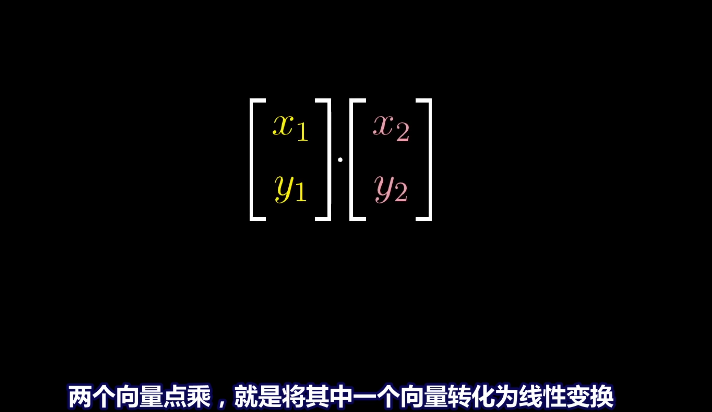
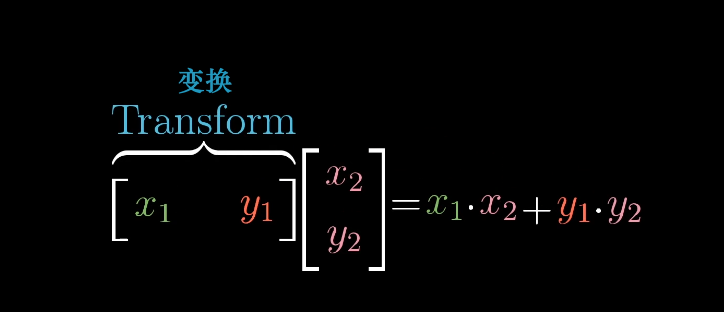