# 如何开发多层感知器模型进行时间序列预测
> 原文: [https://machinelearningmastery.com/how-to-develop-multilayer-perceptron-models-for-time-series-forecasting/](https://machinelearningmastery.com/how-to-develop-multilayer-perceptron-models-for-time-series-forecasting/)
多层感知器(简称 MLP)可应用于时间序列预测。
使用 MLP 进行时间序列预测的一个挑战是准备数据。具体而言,必须将滞后观察平坦化为特征向量。
在本教程中,您将了解如何针对一系列标准时间序列预测问题开发一套 MLP 模型。
本教程的目的是为每种类型的时间序列问题提供每个模型的独立示例,作为模板,您可以根据特定的时间序列预测问题进行复制和调整。
在本教程中,您将了解如何针对一系列标准时间序列预测问题开发一套多层感知器模型。
完成本教程后,您将了解:
* 如何开发单变量时间序列预测的 MLP 模型。
* 如何开发多元时间序列预测的 MLP 模型。
* 如何开发 MLP 模型进行多步时间序列预测。
让我们开始吧。
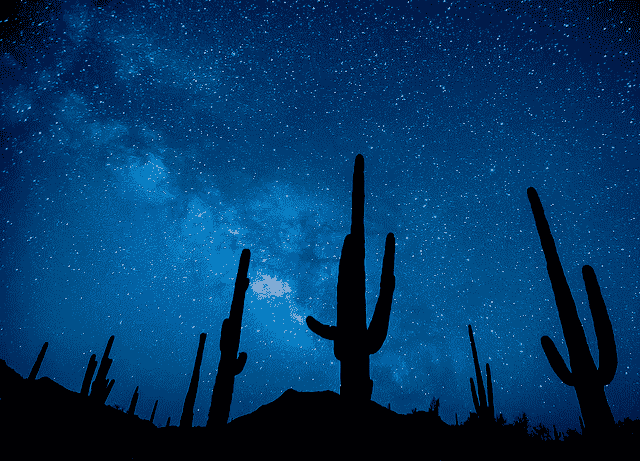
如何开发用于时间序列预测的多层感知器模型
照片由[土地管理局](https://www.flickr.com/photos/mypubliclands/16358796247/),保留一些权利。
## 教程概述
本教程分为四个部分;他们是:
1. 单变量 MLP 模型
2. 多变量 MLP 模型
3. 多步 MLP 模型
4. 多变量多步 MLP 模型
## 单变量 MLP 模型
多层感知器(简称 MLP)可用于模拟单变量时间序列预测问题。
单变量时间序列是由具有时间顺序的单个观察序列组成的数据集,并且需要模型来从过去的一系列观察中学习以预测序列中的下一个值。
本节分为两部分;他们是:
1. 数据准备
2. MLP 模型
### 数据准备
在对单变量系列进行建模之前,必须准备好它。
MLP 模型将学习将过去观察序列作为输入映射到输出观察的函数。因此,必须将观察序列转换为模型可以从中学习的多个示例。
考虑给定的单变量序列:
```py
[10, 20, 30, 40, 50, 60, 70, 80, 90]
```
我们可以将序列划分为多个称为样本的输入/输出模式,其中三个时间步长用作输入,一个时间步长用作正在学习的一步预测的输出。
```py
X, y
10, 20, 30 40
20, 30, 40 50
30, 40, 50 60
...
```
下面的 _split_sequence()_ 函数实现了这种行为,并将给定的单变量序列分成多个样本,其中每个样本具有指定的时间步长,输出是单个时间步长。
```py
# split a univariate sequence into samples
def split_sequence(sequence, n_steps):
X, y = list(), list()
for i in range(len(sequence)):
# find the end of this pattern
end_ix = i + n_steps
# check if we are beyond the sequence
if end_ix > len(sequence)-1:
break
# gather input and output parts of the pattern
seq_x, seq_y = sequence[i:end_ix], sequence[end_ix]
X.append(seq_x)
y.append(seq_y)
return array(X), array(y)
```
我们可以在上面的小型人为数据集上演示这个功能。
下面列出了完整的示例。
```py
# univariate data preparation
from numpy import array
# split a univariate sequence into samples
def split_sequence(sequence, n_steps):
X, y = list(), list()
for i in range(len(sequence)):
# find the end of this pattern
end_ix = i + n_steps
# check if we are beyond the sequence
if end_ix > len(sequence)-1:
break
# gather input and output parts of the pattern
seq_x, seq_y = sequence[i:end_ix], sequence[end_ix]
X.append(seq_x)
y.append(seq_y)
return array(X), array(y)
# define input sequence
raw_seq = [10, 20, 30, 40, 50, 60, 70, 80, 90]
# choose a number of time steps
n_steps = 3
# split into samples
X, y = split_sequence(raw_seq, n_steps)
# summarize the data
for i in range(len(X)):
print(X[i], y[i])
```
运行该示例将单变量系列分成六个样本,其中每个样本具有三个输入时间步长和一个输出时间步长。
```py
[10 20 30] 40
[20 30 40] 50
[30 40 50] 60
[40 50 60] 70
[50 60 70] 80
[60 70 80] 90
```
现在我们已经知道如何准备用于建模的单变量系列,让我们看看开发一个可以学习输入到输出的映射的 MLP 模型。
### MLP 模型
简单的 MLP 模型具有单个隐藏的节点层,以及用于进行预测的输出层。
我们可以如下定义用于单变量时间序列预测的 MLP。
```py
# define model
model = Sequential()
model.add(Dense(100, activation='relu', input_dim=n_steps))
model.add(Dense(1))
model.compile(optimizer='adam', loss='mse')
```
定义中重要的是输入的形状;这就是模型所期望的每个样本在时间步数方面的输入。
输入的时间步数是我们在准备数据集时选择的数字,作为 _split_sequence()_ 函数的参数。
每个样本的输入维度在第一个隐藏层定义的 _input_dim_ 参数中指定。从技术上讲,模型将每个时间步骤视为单独的特征而不是单独的时间步骤。
我们几乎总是有多个样本,因此,模型将期望训练数据的输入组件具有尺寸或形状:
```py
[samples, features]
```
我们在上一节中的 _split_sequence()_ 函数输出 _X_ ,形状 _[样本,特征]_ 准备用于建模。
使用随机梯度下降的有效 [Adam 版本拟合该模型,并使用均方误差或' _mse_ ',损失函数进行优化。](https://machinelearningmastery.com/adam-optimization-algorithm-for-deep-learning/)
定义模型后,我们可以将其放在训练数据集上。
```py
# fit model
model.fit(X, y, epochs=2000, verbose=0)
```
在模型拟合后,我们可以使用它来进行预测。
我们可以通过提供输入来预测序列中的下一个值:
```py
[70, 80, 90]
```
并期望模型预测如下:
```py
[100]
```
模型期望输入形状为 _[样本,特征]_ 为二维,因此,我们必须在进行预测之前重新整形单个输入样本,例如,对于 1 个样本,形状为[1,3]和 3 个时间步骤用作输入功能。
```py
# demonstrate prediction
x_input = array([70, 80, 90])
x_input = x_input.reshape((1, n_steps))
yhat = model.predict(x_input, verbose=0)
```
我们可以将所有这些结合在一起并演示如何为单变量时间序列预测开发 MLP 并进行单一预测。
```py
# univariate mlp example
from numpy import array
from keras.models import Sequential
from keras.layers import Dense
# split a univariate sequence into samples
def split_sequence(sequence, n_steps):
X, y = list(), list()
for i in range(len(sequence)):
# find the end of this pattern
end_ix = i + n_steps
# check if we are beyond the sequence
if end_ix > len(sequence)-1:
break
# gather input and output parts of the pattern
seq_x, seq_y = sequence[i:end_ix], sequence[end_ix]
X.append(seq_x)
y.append(seq_y)
return array(X), array(y)
# define input sequence
raw_seq = [10, 20, 30, 40, 50, 60, 70, 80, 90]
# choose a number of time steps
n_steps = 3
# split into samples
X, y = split_sequence(raw_seq, n_steps)
# define model
model = Sequential()
model.add(Dense(100, activation='relu', input_dim=n_steps))
model.add(Dense(1))
model.compile(optimizer='adam', loss='mse')
# fit model
model.fit(X, y, epochs=2000, verbose=0)
# demonstrate prediction
x_input = array([70, 80, 90])
x_input = x_input.reshape((1, n_steps))
yhat = model.predict(x_input, verbose=0)
print(yhat)
```
运行该示例准备数据,拟合模型并进行预测。
鉴于算法的随机性,您的结果可能会有所不同;尝试运行几次这个例子。
我们可以看到模型预测序列中的下一个值。
```py
[[100.0109]]
```
## 多变量 MLP 模型
多变量时间序列数据是指每个时间步长有多个观察值的数据。
对于多变量时间序列数据,我们可能需要两种主要模型;他们是:
1. 多输入系列。
2. 多个并联系列。
让我们依次看看每一个。
### 多输入系列
问题可能有两个或更多并行输入时间序列和输出时间序列,这取决于输入时间序列。
输入时间序列是平行的,因为每个系列在同一时间步骤都有观察。
我们可以通过两个并行输入时间序列的简单示例来演示这一点,其中输出序列是输入序列的简单添加。
```py
# define input sequence
in_seq1 = array([10, 20, 30, 40, 50, 60, 70, 80, 90])
in_seq2 = array([15, 25, 35, 45, 55, 65, 75, 85, 95])
out_seq = array([in_seq1[i]+in_seq2[i] for i in range(len(in_seq1))])
```
我们可以将这三个数据数组重新整形为单个数据集,其中每一行都是一个时间步,每列是一个单独的时间序列。这是将并行时间序列存储在 CSV 文件中的标准方法。
```py
# convert to [rows, columns] structure
in_seq1 = in_seq1.reshape((len(in_seq1), 1))
in_seq2 = in_seq2.reshape((len(in_seq2), 1))
out_seq = out_seq.reshape((len(out_seq), 1))
# horizontally stack columns
dataset = hstack((in_seq1, in_seq2, out_seq))
```
下面列出了完整的示例。
```py
# multivariate data preparation
from numpy import array
from numpy import hstack
# define input sequence
in_seq1 = array([10, 20, 30, 40, 50, 60, 70, 80, 90])
in_seq2 = array([15, 25, 35, 45, 55, 65, 75, 85, 95])
out_seq = array([in_seq1[i]+in_seq2[i] for i in range(len(in_seq1))])
# convert to [rows, columns] structure
in_seq1 = in_seq1.reshape((len(in_seq1), 1))
in_seq2 = in_seq2.reshape((len(in_seq2), 1))
out_seq = out_seq.reshape((len(out_seq), 1))
# horizontally stack columns
dataset = hstack((in_seq1, in_seq2, out_seq))
print(dataset)
```
运行该示例将打印数据集,每个时间步长为一行,两个输入和一个输出并行时间序列分别为一列。
```py
[[ 10 15 25]
[ 20 25 45]
[ 30 35 65]
[ 40 45 85]
[ 50 55 105]
[ 60 65 125]
[ 70 75 145]
[ 80 85 165]
[ 90 95 185]]
```
与单变量时间序列一样,我们必须将这些数据组织成具有输入和输出样本的样本。
我们需要将数据分成样本,保持两个输入序列的观察顺序。
如果我们选择三个输入时间步长,那么第一个样本将如下所示:
输入:
```py
10, 15
20, 25
30, 35
```
输出:
```py
65
```
也就是说,每个并行系列的前三个时间步长被提供作为模型的输入,并且模型将其与第三时间步骤的输出系列中的值相关联,在这种情况下为 65。
我们可以看到,在将时间序列转换为输入/输出样本以训练模型时,我们将不得不从输出时间序列中丢弃一些值,其中我们在先前时间步骤中没有输入时间序列中的值。反过来,选择输入时间步数的大小将对使用多少训练数据产生重要影响。
我们可以定义一个名为 _split_sequences()_ 的函数,该函数将采用数据集,因为我们已经为时间步长和行定义了并行序列和返回输入/输出样本的列。
```py
# split a multivariate sequence into samples
def split_sequences(sequences, n_steps):
X, y = list(), list()
for i in range(len(sequences)):
# find the end of this pattern
end_ix = i + n_steps
# check if we are beyond the dataset
if end_ix > len(sequences):
break
# gather input and output parts of the pattern
seq_x, seq_y = sequences[i:end_ix, :-1], sequences[end_ix-1, -1]
X.append(seq_x)
y.append(seq_y)
return array(X), array(y)
```
我们可以使用每个输入时间序列的三个时间步长作为输入在我们的数据集上测试此函数。
下面列出了完整的示例。
```py
# multivariate data preparation
from numpy import array
from numpy import hstack
# split a multivariate sequence into samples
def split_sequences(sequences, n_steps):
X, y = list(), list()
for i in range(len(sequences)):
# find the end of this pattern
end_ix = i + n_steps
# check if we are beyond the dataset
if end_ix > len(sequences):
break
# gather input and output parts of the pattern
seq_x, seq_y = sequences[i:end_ix, :-1], sequences[end_ix-1, -1]
X.append(seq_x)
y.append(seq_y)
return array(X), array(y)
# define input sequence
in_seq1 = array([10, 20, 30, 40, 50, 60, 70, 80, 90])
in_seq2 = array([15, 25, 35, 45, 55, 65, 75, 85, 95])
out_seq = array([in_seq1[i]+in_seq2[i] for i in range(len(in_seq1))])
# convert to [rows, columns] structure
in_seq1 = in_seq1.reshape((len(in_seq1), 1))
in_seq2 = in_seq2.reshape((len(in_seq2), 1))
out_seq = out_seq.reshape((len(out_seq), 1))
# horizontally stack columns
dataset = hstack((in_seq1, in_seq2, out_seq))
# choose a number of time steps
n_steps = 3
# convert into input/output
X, y = split_sequences(dataset, n_steps)
print(X.shape, y.shape)
# summarize the data
for i in range(len(X)):
print(X[i], y[i])
```
首先运行该示例将打印 X 和 y 组件的形状。
我们可以看到 X 组件具有三维结构。
第一个维度是样本数,在本例中为 7.第二个维度是每个样本的时间步数,在这种情况下为 3,即为函数指定的值。最后,最后一个维度指定并行时间序列的数量或变量的数量,在这种情况下,两个并行序列为 2。
然后我们可以看到每个样本的输入和输出都被打印出来,显示了两个输入序列中每个样本的三个时间步长以及每个样本的相关输出。
```py
(7, 3, 2) (7,)
[[10 15]
[20 25]
[30 35]] 65
[[20 25]
[30 35]
[40 45]] 85
[[30 35]
[40 45]
[50 55]] 105
[[40 45]
[50 55]
[60 65]] 125
[[50 55]
[60 65]
[70 75]] 145
[[60 65]
[70 75]
[80 85]] 165
[[70 75]
[80 85]
[90 95]] 185
```
在我们可以在这些数据上拟合 MLP 之前,我们必须平整输入样本的形状。
MLP 要求每个样本的输入部分的形状是向量。使用多变量输入,我们将有多个向量,每个时间步长一个。
我们可以展平每个输入样本的时间结构,以便:
```py
[[10 15]
[20 25]
[30 35]]
```
变为:
```py
[10, 15, 20, 25, 30, 35]
```
首先,我们可以计算每个输入向量的长度,作为时间步数乘以要素数或时间序列数。然后我们可以使用此向量大小来重塑输入。
```py
# flatten input
n_input = X.shape[1] * X.shape[2]
X = X.reshape((X.shape[0], n_input))
```
我们现在可以为多变量输入定义 MLP 模型,其中向量长度用于输入维度参数。
```py
# define model
model = Sequential()
model.add(Dense(100, activation='relu', input_dim=n_input))
model.add(Dense(1))
model.compile(optimizer='adam', loss='mse')
```
在进行预测时,模型需要两个输入时间序列的三个时间步长。
我们可以预测输出系列中的下一个值,证明输入值:
```py
80, 85
90, 95
100, 105
```
具有 3 个时间步长和 2 个变量的 1 个样本的形状将是[1,3,2]。我们必须再次将其重塑为 1 个样本,其中包含 6 个元素的向量或[1,6]
我们希望序列中的下一个值为 100 + 105 或 205。
```py
# demonstrate prediction
x_input = array([[80, 85], [90, 95], [100, 105]])
x_input = x_input.reshape((1, n_input))
yhat = model.predict(x_input, verbose=0)
```
下面列出了完整的示例。
```py
# multivariate mlp example
from numpy import array
from numpy import hstack
from keras.models import Sequential
from keras.layers import Dense
# split a multivariate sequence into samples
def split_sequences(sequences, n_steps):
X, y = list(), list()
for i in range(len(sequences)):
# find the end of this pattern
end_ix = i + n_steps
# check if we are beyond the dataset
if end_ix > len(sequences):
break
# gather input and output parts of the pattern
seq_x, seq_y = sequences[i:end_ix, :-1], sequences[end_ix-1, -1]
X.append(seq_x)
y.append(seq_y)
return array(X), array(y)
# define input sequence
in_seq1 = array([10, 20, 30, 40, 50, 60, 70, 80, 90])
in_seq2 = array([15, 25, 35, 45, 55, 65, 75, 85, 95])
out_seq = array([in_seq1[i]+in_seq2[i] for i in range(len(in_seq1))])
# convert to [rows, columns] structure
in_seq1 = in_seq1.reshape((len(in_seq1), 1))
in_seq2 = in_seq2.reshape((len(in_seq2), 1))
out_seq = out_seq.reshape((len(out_seq), 1))
# horizontally stack columns
dataset = hstack((in_seq1, in_seq2, out_seq))
# choose a number of time steps
n_steps = 3
# convert into input/output
X, y = split_sequences(dataset, n_steps)
# flatten input
n_input = X.shape[1] * X.shape[2]
X = X.reshape((X.shape[0], n_input))
# define model
model = Sequential()
model.add(Dense(100, activation='relu', input_dim=n_input))
model.add(Dense(1))
model.compile(optimizer='adam', loss='mse')
# fit model
model.fit(X, y, epochs=2000, verbose=0)
# demonstrate prediction
x_input = array([[80, 85], [90, 95], [100, 105]])
x_input = x_input.reshape((1, n_input))
yhat = model.predict(x_input, verbose=0)
print(yhat)
```
运行该示例准备数据,拟合模型并进行预测。
```py
[[205.04436]]
```
还有另一种更精细的方法来模拟问题。
每个输入序列可以由单独的 MLP 处理,并且可以在对输出序列进行预测之前组合这些子模型中的每一个的输出。
我们可以将其称为多头输入 MLP 模型。根据正在建模的问题的具体情况,它可以提供更大的灵活性或更好的表现。
可以使用 [Keras 功能 API](https://machinelearningmastery.com/keras-functional-api-deep-learning/) 在 Keras 中定义此类型的模型。
首先,我们可以将第一个输入模型定义为 MLP,其输入层需要具有 _n_steps_ 特征的向量。
```py
# first input model
visible1 = Input(shape=(n_steps,))
dense1 = Dense(100, activation='relu')(visible1)
```
我们可以以相同的方式定义第二个输入子模型。
```py
# second input model
visible2 = Input(shape=(n_steps,))
dense2 = Dense(100, activation='relu')(visible2)
```
既然已经定义了两个输入子模型,我们可以将每个模型的输出合并为一个长向量,可以在对输出序列进行预测之前对其进行解释。
```py
# merge input models
merge = concatenate([dense1, dense2])
output = Dense(1)(merge)
```
然后我们可以将输入和输出联系在一起。
```py
model = Model(inputs=[visible1, visible2], outputs=output)
```
下图提供了该模型外观的示意图,包括每层输入和输出的形状。
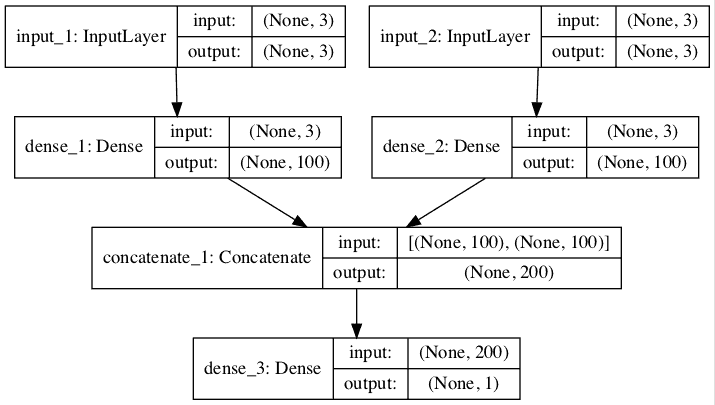
多元时间序列预测的多头 MLP 图
此模型要求输入作为两个元素的列表提供,其中列表中的每个元素包含一个子模型的数据。
为了实现这一点,我们可以将 3D 输入数据分成两个独立的输入数据阵列:即从一个形状为[7,3,2]的阵列到两个形状为[7,3]的 2D 阵列
```py
# separate input data
X1 = X[:, :, 0]
X2 = X[:, :, 1]
```
然后可以提供这些数据以适合模型。
```py
# fit model
model.fit([X1, X2], y, epochs=2000, verbose=0)
```
类似地,我们必须在进行单个一步预测时将单个样本的数据准备为两个单独的二维数组。
```py
x_input = array([[80, 85], [90, 95], [100, 105]])
x1 = x_input[:, 0].reshape((1, n_steps))
x2 = x_input[:, 1].reshape((1, n_steps))
```
我们可以将所有这些结合在一起;下面列出了完整的示例。
```py
# multivariate mlp example
from numpy import array
from numpy import hstack
from keras.models import Model
from keras.layers import Input
from keras.layers import Dense
from keras.layers.merge import concatenate
# split a multivariate sequence into samples
def split_sequences(sequences, n_steps):
X, y = list(), list()
for i in range(len(sequences)):
# find the end of this pattern
end_ix = i + n_steps
# check if we are beyond the dataset
if end_ix > len(sequences):
break
# gather input and output parts of the pattern
seq_x, seq_y = sequences[i:end_ix, :-1], sequences[end_ix-1, -1]
X.append(seq_x)
y.append(seq_y)
return array(X), array(y)
# define input sequence
in_seq1 = array([10, 20, 30, 40, 50, 60, 70, 80, 90])
in_seq2 = array([15, 25, 35, 45, 55, 65, 75, 85, 95])
out_seq = array([in_seq1[i]+in_seq2[i] for i in range(len(in_seq1))])
# convert to [rows, columns] structure
in_seq1 = in_seq1.reshape((len(in_seq1), 1))
in_seq2 = in_seq2.reshape((len(in_seq2), 1))
out_seq = out_seq.reshape((len(out_seq), 1))
# horizontally stack columns
dataset = hstack((in_seq1, in_seq2, out_seq))
# choose a number of time steps
n_steps = 3
# convert into input/output
X, y = split_sequences(dataset, n_steps)
# separate input data
X1 = X[:, :, 0]
X2 = X[:, :, 1]
# first input model
visible1 = Input(shape=(n_steps,))
dense1 = Dense(100, activation='relu')(visible1)
# second input model
visible2 = Input(shape=(n_steps,))
dense2 = Dense(100, activation='relu')(visible2)
# merge input models
merge = concatenate([dense1, dense2])
output = Dense(1)(merge)
model = Model(inputs=[visible1, visible2], outputs=output)
model.compile(optimizer='adam', loss='mse')
# fit model
model.fit([X1, X2], y, epochs=2000, verbose=0)
# demonstrate prediction
x_input = array([[80, 85], [90, 95], [100, 105]])
x1 = x_input[:, 0].reshape((1, n_steps))
x2 = x_input[:, 1].reshape((1, n_steps))
yhat = model.predict([x1, x2], verbose=0)
print(yhat)
```
运行该示例准备数据,拟合模型并进行预测。
```py
[[206.05022]]
```
### 多个并联系列
另一个时间序列问题是存在多个并行时间序列并且必须为每个时间序列预测值的情况。
例如,给定上一节的数据:
```py
[[ 10 15 25]
[ 20 25 45]
[ 30 35 65]
[ 40 45 85]
[ 50 55 105]
[ 60 65 125]
[ 70 75 145]
[ 80 85 165]
[ 90 95 185]]
```
我们可能想要预测下一个时间步的三个时间序列中的每一个的值。
这可以称为多变量预测。
同样,必须将数据分成输入/输出样本以训练模型。
该数据集的第一个示例是:
输入:
```py
10, 15, 25
20, 25, 45
30, 35, 65
```
输出:
```py
40, 45, 85
```
下面的 _split_sequences()_ 函数将分割多个并行时间序列,其中时间步长为行,每列一个系列为所需的输入/输出形状。
```py
# split a multivariate sequence into samples
def split_sequences(sequences, n_steps):
X, y = list(), list()
for i in range(len(sequences)):
# find the end of this pattern
end_ix = i + n_steps
# check if we are beyond the dataset
if end_ix > len(sequences)-1:
break
# gather input and output parts of the pattern
seq_x, seq_y = sequences[i:end_ix, :], sequences[end_ix, :]
X.append(seq_x)
y.append(seq_y)
return array(X), array(y)
```
我们可以在人为的问题上证明这一点;下面列出了完整的示例。
```py
# multivariate output data prep
from numpy import array
from numpy import hstack
# split a multivariate sequence into samples
def split_sequences(sequences, n_steps):
X, y = list(), list()
for i in range(len(sequences)):
# find the end of this pattern
end_ix = i + n_steps
# check if we are beyond the dataset
if end_ix > len(sequences)-1:
break
# gather input and output parts of the pattern
seq_x, seq_y = sequences[i:end_ix, :], sequences[end_ix, :]
X.append(seq_x)
y.append(seq_y)
return array(X), array(y)
# define input sequence
in_seq1 = array([10, 20, 30, 40, 50, 60, 70, 80, 90])
in_seq2 = array([15, 25, 35, 45, 55, 65, 75, 85, 95])
out_seq = array([in_seq1[i]+in_seq2[i] for i in range(len(in_seq1))])
# convert to [rows, columns] structure
in_seq1 = in_seq1.reshape((len(in_seq1), 1))
in_seq2 = in_seq2.reshape((len(in_seq2), 1))
out_seq = out_seq.reshape((len(out_seq), 1))
# horizontally stack columns
dataset = hstack((in_seq1, in_seq2, out_seq))
# choose a number of time steps
n_steps = 3
# convert into input/output
X, y = split_sequences(dataset, n_steps)
print(X.shape, y.shape)
# summarize the data
for i in range(len(X)):
print(X[i], y[i])
```
首先运行该实例打印制备的 _X_ 和 _y_ 组分的形状。
_X_ 的形状是三维的,包括样品的数量(6),每个样品选择的时间步数(3),以及平行时间序列或特征的数量(3)。
_y_ 的形状是二维的,正如我们对样本数(6)和每个样本预测的时间变量数(3)所预期的那样。
然后,打印每个样本,显示每个样本的输入和输出分量。
```py
(6, 3, 3) (6, 3)
[[10 15 25]
[20 25 45]
[30 35 65]] [40 45 85]
[[20 25 45]
[30 35 65]
[40 45 85]] [ 50 55 105]
[[ 30 35 65]
[ 40 45 85]
[ 50 55 105]] [ 60 65 125]
[[ 40 45 85]
[ 50 55 105]
[ 60 65 125]] [ 70 75 145]
[[ 50 55 105]
[ 60 65 125]
[ 70 75 145]] [ 80 85 165]
[[ 60 65 125]
[ 70 75 145]
[ 80 85 165]] [ 90 95 185]
```
我们现在准备在这些数据上安装 MLP 模型。
与之前的多变量输入情况一样,我们必须将输入数据样本的三维结构展平为[_ 样本,特征 _]的二维结构,其中滞后观察被模型视为特征。
```py
# flatten input
n_input = X.shape[1] * X.shape[2]
X = X.reshape((X.shape[0], n_input))
```
模型输出将是一个向量,三个不同时间序列中的每一个都有一个元素。
```py
n_output = y.shape[1]
```
我们现在可以定义我们的模型,使用输入层的展平向量长度和进行预测时的向量长度作为向量长度。
```py
# define model
model = Sequential()
model.add(Dense(100, activation='relu', input_dim=n_input))
model.add(Dense(n_output))
model.compile(optimizer='adam', loss='mse')
```
我们可以通过为每个系列提供三个时间步长的输入来预测三个并行系列中的每一个的下一个值。
```py
70, 75, 145
80, 85, 165
90, 95, 185
```
用于进行单个预测的输入的形状必须是 1 个样本,3 个时间步长和 3 个特征,或者[1,3,3]。同样,我们可以将其展平为[1,6]以满足模型的期望。
我们希望向量输出为:
```py
[100, 105, 205]
```
```py
# demonstrate prediction
x_input = array([[70,75,145], [80,85,165], [90,95,185]])
x_input = x_input.reshape((1, n_input))
yhat = model.predict(x_input, verbose=0)
```
我们可以将所有这些结合在一起并演示下面的多变量输出时间序列预测的 MLP。
```py
# multivariate output mlp example
from numpy import array
from numpy import hstack
from keras.models import Sequential
from keras.layers import Dense
# split a multivariate sequence into samples
def split_sequences(sequences, n_steps):
X, y = list(), list()
for i in range(len(sequences)):
# find the end of this pattern
end_ix = i + n_steps
# check if we are beyond the dataset
if end_ix > len(sequences)-1:
break
# gather input and output parts of the pattern
seq_x, seq_y = sequences[i:end_ix, :], sequences[end_ix, :]
X.append(seq_x)
y.append(seq_y)
return array(X), array(y)
# define input sequence
in_seq1 = array([10, 20, 30, 40, 50, 60, 70, 80, 90])
in_seq2 = array([15, 25, 35, 45, 55, 65, 75, 85, 95])
out_seq = array([in_seq1[i]+in_seq2[i] for i in range(len(in_seq1))])
# convert to [rows, columns] structure
in_seq1 = in_seq1.reshape((len(in_seq1), 1))
in_seq2 = in_seq2.reshape((len(in_seq2), 1))
out_seq = out_seq.reshape((len(out_seq), 1))
# horizontally stack columns
dataset = hstack((in_seq1, in_seq2, out_seq))
# choose a number of time steps
n_steps = 3
# convert into input/output
X, y = split_sequences(dataset, n_steps)
# flatten input
n_input = X.shape[1] * X.shape[2]
X = X.reshape((X.shape[0], n_input))
n_output = y.shape[1]
# define model
model = Sequential()
model.add(Dense(100, activation='relu', input_dim=n_input))
model.add(Dense(n_output))
model.compile(optimizer='adam', loss='mse')
# fit model
model.fit(X, y, epochs=2000, verbose=0)
# demonstrate prediction
x_input = array([[70,75,145], [80,85,165], [90,95,185]])
x_input = x_input.reshape((1, n_input))
yhat = model.predict(x_input, verbose=0)
print(yhat)
```
运行该示例准备数据,拟合模型并进行预测。
```py
[[100.95039 107.541306 206.81033 ]]
```
与多输入系列一样,还有另一种更精细的方法来模拟问题。
每个输出系列都可以由单独的输出 MLP 模型处理。
我们可以将其称为多输出 MLP 模型。根据正在建模的问题的具体情况,它可以提供更大的灵活性或更好的表现。
可以使用 [Keras 功能 API](https://machinelearningmastery.com/keras-functional-api-deep-learning/) 在 Keras 中定义此类型的模型。
首先,我们可以将输入模型定义为 MLP,其输入层需要平坦的特征向量。
```py
# define model
visible = Input(shape=(n_input,))
dense = Dense(100, activation='relu')(visible)
```
然后,我们可以为我们希望预测的三个系列中的每一个定义一个输出层,其中每个输出子模型将预测单个时间步长。
```py
# define output 1
output1 = Dense(1)(dense)
# define output 2
output2 = Dense(1)(dense)
# define output 2
output3 = Dense(1)(dense)
```
然后,我们可以将输入和输出层组合到一个模型中。
```py
# tie together
model = Model(inputs=visible, outputs=[output1, output2, output3])
model.compile(optimizer='adam', loss='mse')
```
为了使模型架构清晰,下面的示意图清楚地显示了模型的三个独立输出层以及每个层的输入和输出形状。
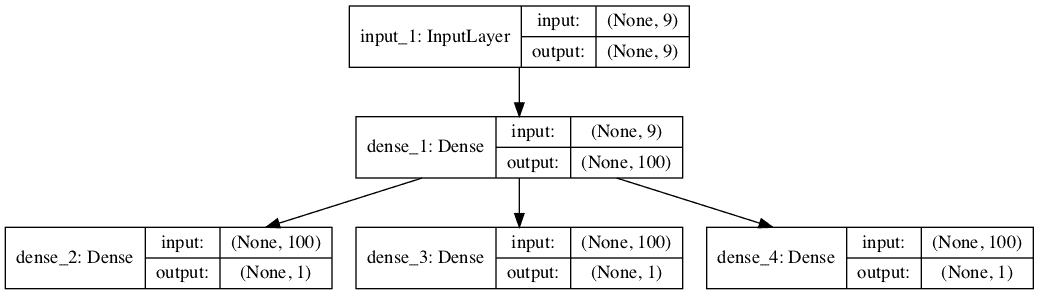
多元时间序列预测的多输出 MLP 图
在训练模型时,每个样本需要三个独立的输出阵列。
我们可以通过将具有形状[7,3]的输出训练数据转换为具有形状[7,1]的三个阵列来实现这一点。
```py
# separate output
y1 = y[:, 0].reshape((y.shape[0], 1))
y2 = y[:, 1].reshape((y.shape[0], 1))
y3 = y[:, 2].reshape((y.shape[0], 1))
```
可以在训练期间将这些阵列提供给模型。
```py
# fit model
model.fit(X, [y1,y2,y3], epochs=2000, verbose=0)
```
将所有这些结合在一起,下面列出了完整的示例。
```py
# multivariate output mlp example
from numpy import array
from numpy import hstack
from keras.models import Model
from keras.layers import Input
from keras.layers import Dense
# split a multivariate sequence into samples
def split_sequences(sequences, n_steps):
X, y = list(), list()
for i in range(len(sequences)):
# find the end of this pattern
end_ix = i + n_steps
# check if we are beyond the dataset
if end_ix > len(sequences)-1:
break
# gather input and output parts of the pattern
seq_x, seq_y = sequences[i:end_ix, :], sequences[end_ix, :]
X.append(seq_x)
y.append(seq_y)
return array(X), array(y)
# define input sequence
in_seq1 = array([10, 20, 30, 40, 50, 60, 70, 80, 90])
in_seq2 = array([15, 25, 35, 45, 55, 65, 75, 85, 95])
out_seq = array([in_seq1[i]+in_seq2[i] for i in range(len(in_seq1))])
# convert to [rows, columns] structure
in_seq1 = in_seq1.reshape((len(in_seq1), 1))
in_seq2 = in_seq2.reshape((len(in_seq2), 1))
out_seq = out_seq.reshape((len(out_seq), 1))
# horizontally stack columns
dataset = hstack((in_seq1, in_seq2, out_seq))
# choose a number of time steps
n_steps = 3
# convert into input/output
X, y = split_sequences(dataset, n_steps)
# flatten input
n_input = X.shape[1] * X.shape[2]
X = X.reshape((X.shape[0], n_input))
# separate output
y1 = y[:, 0].reshape((y.shape[0], 1))
y2 = y[:, 1].reshape((y.shape[0], 1))
y3 = y[:, 2].reshape((y.shape[0], 1))
# define model
visible = Input(shape=(n_input,))
dense = Dense(100, activation='relu')(visible)
# define output 1
output1 = Dense(1)(dense)
# define output 2
output2 = Dense(1)(dense)
# define output 2
output3 = Dense(1)(dense)
# tie together
model = Model(inputs=visible, outputs=[output1, output2, output3])
model.compile(optimizer='adam', loss='mse')
# fit model
model.fit(X, [y1,y2,y3], epochs=2000, verbose=0)
# demonstrate prediction
x_input = array([[70,75,145], [80,85,165], [90,95,185]])
x_input = x_input.reshape((1, n_input))
yhat = model.predict(x_input, verbose=0)
print(yhat)
```
运行该示例准备数据,拟合模型并进行预测。
```py
[array([[100.86121]], dtype=float32),
array([[105.14738]], dtype=float32),
array([[205.97507]], dtype=float32)]
```
## 多步 MLP 模型
实际上,MLP 模型在预测表示不同输出变量的向量输出(如前例中所示)或表示一个变量的多个时间步长的向量输出方面几乎没有差别。
然而,训练数据的编制方式存在细微而重要的差异。在本节中,我们将演示使用向量模型开发多步预测模型的情况。
在我们查看模型的细节之前,让我们首先看一下多步预测的数据准备。
### 数据准备
与一步预测一样,用于多步时间序列预测的时间序列必须分为带有输入和输出组件的样本。
输入和输出组件都将包含多个时间步长,并且可以具有或不具有相同数量的步骤。
例如,给定单变量时间序列:
```py
[10, 20, 30, 40, 50, 60, 70, 80, 90]
```
我们可以使用最后三个时间步作为输入并预测接下来的两个时间步。
第一个样本如下:
输入:
```py
[10, 20, 30]
```
输出:
```py
[40, 50]
```
下面的 _split_sequence()_ 函数实现了这种行为,并将给定的单变量时间序列分割为具有指定数量的输入和输出时间步长的样本。
```py
# split a univariate sequence into samples
def split_sequence(sequence, n_steps_in, n_steps_out):
X, y = list(), list()
for i in range(len(sequence)):
# find the end of this pattern
end_ix = i + n_steps_in
out_end_ix = end_ix + n_steps_out
# check if we are beyond the sequence
if out_end_ix > len(sequence):
break
# gather input and output parts of the pattern
seq_x, seq_y = sequence[i:end_ix], sequence[end_ix:out_end_ix]
X.append(seq_x)
y.append(seq_y)
return array(X), array(y)
```
我们可以在小型设计数据集上演示此功能。
下面列出了完整的示例。
```py
# multi-step data preparation
from numpy import array
# split a univariate sequence into samples
def split_sequence(sequence, n_steps_in, n_steps_out):
X, y = list(), list()
for i in range(len(sequence)):
# find the end of this pattern
end_ix = i + n_steps_in
out_end_ix = end_ix + n_steps_out
# check if we are beyond the sequence
if out_end_ix > len(sequence):
break
# gather input and output parts of the pattern
seq_x, seq_y = sequence[i:end_ix], sequence[end_ix:out_end_ix]
X.append(seq_x)
y.append(seq_y)
return array(X), array(y)
# define input sequence
raw_seq = [10, 20, 30, 40, 50, 60, 70, 80, 90]
# choose a number of time steps
n_steps_in, n_steps_out = 3, 2
# split into samples
X, y = split_sequence(raw_seq, n_steps_in, n_steps_out)
# summarize the data
for i in range(len(X)):
print(X[i], y[i])
```
运行该示例将单变量系列拆分为输入和输出时间步骤,并打印每个系列的输入和输出组件。
```py
[10 20 30] [40 50]
[20 30 40] [50 60]
[30 40 50] [60 70]
[40 50 60] [70 80]
[50 60 70] [80 90]
```
既然我们知道如何为多步预测准备数据,那么让我们看一下可以学习这种映射的 MLP 模型。
### 向量输出模型
MLP 可以直接输出向量,可以解释为多步预测。
在前一节中看到这种方法是每个输出时间序列的一个时间步骤被预测为向量。
通过 _n_steps_in_ 和 _n_steps_out_ 变量中指定的输入和输出步数,我们可以定义一个多步骤时间序列预测模型。
```py
# define model
model = Sequential()
model.add(Dense(100, activation='relu', input_dim=n_steps_in))
model.add(Dense(n_steps_out))
model.compile(optimizer='adam', loss='mse')
```
该模型可以对单个样本进行预测。我们可以通过提供输入来预测数据集末尾之后的下两个步骤:
```py
[70, 80, 90]
```
我们希望预测的输出为:
```py
[100, 110]
```
正如模型所预期的那样,进行预测时输入数据的单个样本的形状对于输入和单个特征的 1 个样本和 3 个时间步长(特征)必须是[1,3]。
```py
# demonstrate prediction
x_input = array([70, 80, 90])
x_input = x_input.reshape((1, n_steps_in))
yhat = model.predict(x_input, verbose=0)
```
将所有这些结合在一起,下面列出了具有单变量时间序列的多步骤预测的 MLP。
```py
# univariate multi-step vector-output mlp example
from numpy import array
from keras.models import Sequential
from keras.layers import Dense
# split a univariate sequence into samples
def split_sequence(sequence, n_steps_in, n_steps_out):
X, y = list(), list()
for i in range(len(sequence)):
# find the end of this pattern
end_ix = i + n_steps_in
out_end_ix = end_ix + n_steps_out
# check if we are beyond the sequence
if out_end_ix > len(sequence):
break
# gather input and output parts of the pattern
seq_x, seq_y = sequence[i:end_ix], sequence[end_ix:out_end_ix]
X.append(seq_x)
y.append(seq_y)
return array(X), array(y)
# define input sequence
raw_seq = [10, 20, 30, 40, 50, 60, 70, 80, 90]
# choose a number of time steps
n_steps_in, n_steps_out = 3, 2
# split into samples
X, y = split_sequence(raw_seq, n_steps_in, n_steps_out)
# define model
model = Sequential()
model.add(Dense(100, activation='relu', input_dim=n_steps_in))
model.add(Dense(n_steps_out))
model.compile(optimizer='adam', loss='mse')
# fit model
model.fit(X, y, epochs=2000, verbose=0)
# demonstrate prediction
x_input = array([70, 80, 90])
x_input = x_input.reshape((1, n_steps_in))
yhat = model.predict(x_input, verbose=0)
print(yhat)
```
运行示例预测并打印序列中的后两个时间步骤。
```py
[[102.572365 113.88405 ]]
```
## 多变量多步 MLP 模型
在前面的部分中,我们研究了单变量,多变量和多步骤时间序列预测。
可以混合和匹配到目前为止针对不同问题呈现的不同类型的 MLP 模型。这也适用于涉及多变量和多步预测的时间序列预测问题,但它可能更具挑战性,特别是在准备数据和定义模型的输入和输出的形状时。
在本节中,我们将以多变量多步骤时间序列预测的数据准备和建模的简短示例作为模板来缓解这一挑战,具体来说:
1. 多输入多步输出。
2. 多个并行输入和多步输出。
也许最大的绊脚石是准备数据,所以这是我们关注的重点。
### 多输入多步输出
存在多变量时间序列预测问题,其中输出序列是分开的但取决于输入时间序列,并且输出序列需要多个时间步长。
例如,考虑前一部分的多变量时间序列:
```py
[[ 10 15 25]
[ 20 25 45]
[ 30 35 65]
[ 40 45 85]
[ 50 55 105]
[ 60 65 125]
[ 70 75 145]
[ 80 85 165]
[ 90 95 185]]
```
我们可以使用两个输入时间序列中的每一个的三个先前时间步骤来预测输出时间序列的两个时间步长。
输入:
```py
10, 15
20, 25
30, 35
```
输出:
```py
65
85
```
下面的 _split_sequences()_ 函数实现了这种行为。
```py
# split a multivariate sequence into samples
def split_sequences(sequences, n_steps_in, n_steps_out):
X, y = list(), list()
for i in range(len(sequences)):
# find the end of this pattern
end_ix = i + n_steps_in
out_end_ix = end_ix + n_steps_out-1
# check if we are beyond the dataset
if out_end_ix > len(sequences):
break
# gather input and output parts of the pattern
seq_x, seq_y = sequences[i:end_ix, :-1], sequences[end_ix-1:out_end_ix, -1]
X.append(seq_x)
y.append(seq_y)
return array(X), array(y)
```
我们可以在我们设计的数据集上证明这一点。下面列出了完整的示例。
```py
# multivariate multi-step data preparation
from numpy import array
from numpy import hstack
# split a multivariate sequence into samples
def split_sequences(sequences, n_steps_in, n_steps_out):
X, y = list(), list()
for i in range(len(sequences)):
# find the end of this pattern
end_ix = i + n_steps_in
out_end_ix = end_ix + n_steps_out-1
# check if we are beyond the dataset
if out_end_ix > len(sequences):
break
# gather input and output parts of the pattern
seq_x, seq_y = sequences[i:end_ix, :-1], sequences[end_ix-1:out_end_ix, -1]
X.append(seq_x)
y.append(seq_y)
return array(X), array(y)
# define input sequence
in_seq1 = array([10, 20, 30, 40, 50, 60, 70, 80, 90])
in_seq2 = array([15, 25, 35, 45, 55, 65, 75, 85, 95])
out_seq = array([in_seq1[i]+in_seq2[i] for i in range(len(in_seq1))])
# convert to [rows, columns] structure
in_seq1 = in_seq1.reshape((len(in_seq1), 1))
in_seq2 = in_seq2.reshape((len(in_seq2), 1))
out_seq = out_seq.reshape((len(out_seq), 1))
# horizontally stack columns
dataset = hstack((in_seq1, in_seq2, out_seq))
# choose a number of time steps
n_steps_in, n_steps_out = 3, 2
# convert into input/output
X, y = split_sequences(dataset, n_steps_in, n_steps_out)
print(X.shape, y.shape)
# summarize the data
for i in range(len(X)):
print(X[i], y[i])
```
首先运行该示例打印准备好的训练数据的形状。
我们可以看到样本的输入部分的形状是三维的,由六个样本组成,具有三个时间步长和两个输入时间序列的两个变量。
样本的输出部分对于六个样本是二维的,并且每个样本的两个时间步长是预测的。
然后打印制备的样品以确认数据是按照我们指定的方式制备的。
```py
(6, 3, 2) (6, 2)
[[10 15]
[20 25]
[30 35]] [65 85]
[[20 25]
[30 35]
[40 45]] [ 85 105]
[[30 35]
[40 45]
[50 55]] [105 125]
[[40 45]
[50 55]
[60 65]] [125 145]
[[50 55]
[60 65]
[70 75]] [145 165]
[[60 65]
[70 75]
[80 85]] [165 185]
```
我们现在可以使用向量输出开发用于多步预测的 MLP 模型。
下面列出了完整的示例。
```py
# multivariate multi-step mlp example
from numpy import array
from numpy import hstack
from keras.models import Sequential
from keras.layers import Dense
# split a multivariate sequence into samples
def split_sequences(sequences, n_steps_in, n_steps_out):
X, y = list(), list()
for i in range(len(sequences)):
# find the end of this pattern
end_ix = i + n_steps_in
out_end_ix = end_ix + n_steps_out-1
# check if we are beyond the dataset
if out_end_ix > len(sequences):
break
# gather input and output parts of the pattern
seq_x, seq_y = sequences[i:end_ix, :-1], sequences[end_ix-1:out_end_ix, -1]
X.append(seq_x)
y.append(seq_y)
return array(X), array(y)
# define input sequence
in_seq1 = array([10, 20, 30, 40, 50, 60, 70, 80, 90])
in_seq2 = array([15, 25, 35, 45, 55, 65, 75, 85, 95])
out_seq = array([in_seq1[i]+in_seq2[i] for i in range(len(in_seq1))])
# convert to [rows, columns] structure
in_seq1 = in_seq1.reshape((len(in_seq1), 1))
in_seq2 = in_seq2.reshape((len(in_seq2), 1))
out_seq = out_seq.reshape((len(out_seq), 1))
# horizontally stack columns
dataset = hstack((in_seq1, in_seq2, out_seq))
# choose a number of time steps
n_steps_in, n_steps_out = 3, 2
# convert into input/output
X, y = split_sequences(dataset, n_steps_in, n_steps_out)
# flatten input
n_input = X.shape[1] * X.shape[2]
X = X.reshape((X.shape[0], n_input))
# define model
model = Sequential()
model.add(Dense(100, activation='relu', input_dim=n_input))
model.add(Dense(n_steps_out))
model.compile(optimizer='adam', loss='mse')
# fit model
model.fit(X, y, epochs=2000, verbose=0)
# demonstrate prediction
x_input = array([[70, 75], [80, 85], [90, 95]])
x_input = x_input.reshape((1, n_input))
yhat = model.predict(x_input, verbose=0)
print(yhat)
```
运行该示例适合模型并预测输出序列的下两个时间步骤超出数据集。
我们希望接下来的两个步骤是[185,205]。
这是一个具有挑战性的问题框架,数据非常少,模型的任意配置版本也很接近。
```py
[[186.53822 208.41725]]
```
### 多个并行输入和多步输出
并行时间序列的问题可能需要预测每个时间序列的多个时间步长。
例如,考虑前一部分的多变量时间序列:
```py
[[ 10 15 25]
[ 20 25 45]
[ 30 35 65]
[ 40 45 85]
[ 50 55 105]
[ 60 65 125]
[ 70 75 145]
[ 80 85 165]
[ 90 95 185]]
```
我们可以使用三个时间序列中的每一个的最后三个步骤作为模型的输入,并预测三个时间序列中的每一个的下一个时间步长作为输出。
训练数据集中的第一个样本如下。
输入:
```py
10, 15, 25
20, 25, 45
30, 35, 65
```
输出:
```py
40, 45, 85
50, 55, 105
```
下面的 _split_sequences()_ 函数实现了这种行为。
```py
# split a multivariate sequence into samples
def split_sequences(sequences, n_steps_in, n_steps_out):
X, y = list(), list()
for i in range(len(sequences)):
# find the end of this pattern
end_ix = i + n_steps_in
out_end_ix = end_ix + n_steps_out
# check if we are beyond the dataset
if out_end_ix > len(sequences):
break
# gather input and output parts of the pattern
seq_x, seq_y = sequences[i:end_ix, :], sequences[end_ix:out_end_ix, :]
X.append(seq_x)
y.append(seq_y)
return array(X), array(y)
```
我们可以在小型设计数据集上演示此功能。
下面列出了完整的示例。
```py
# multivariate multi-step data preparation
from numpy import array
from numpy import hstack
# split a multivariate sequence into samples
def split_sequences(sequences, n_steps_in, n_steps_out):
X, y = list(), list()
for i in range(len(sequences)):
# find the end of this pattern
end_ix = i + n_steps_in
out_end_ix = end_ix + n_steps_out
# check if we are beyond the dataset
if out_end_ix > len(sequences):
break
# gather input and output parts of the pattern
seq_x, seq_y = sequences[i:end_ix, :], sequences[end_ix:out_end_ix, :]
X.append(seq_x)
y.append(seq_y)
return array(X), array(y)
# define input sequence
in_seq1 = array([10, 20, 30, 40, 50, 60, 70, 80, 90])
in_seq2 = array([15, 25, 35, 45, 55, 65, 75, 85, 95])
out_seq = array([in_seq1[i]+in_seq2[i] for i in range(len(in_seq1))])
# convert to [rows, columns] structure
in_seq1 = in_seq1.reshape((len(in_seq1), 1))
in_seq2 = in_seq2.reshape((len(in_seq2), 1))
out_seq = out_seq.reshape((len(out_seq), 1))
# horizontally stack columns
dataset = hstack((in_seq1, in_seq2, out_seq))
# choose a number of time steps
n_steps_in, n_steps_out = 3, 2
# convert into input/output
X, y = split_sequences(dataset, n_steps_in, n_steps_out)
print(X.shape, y.shape)
# summarize the data
for i in range(len(X)):
print(X[i], y[i])
```
首先运行该示例打印准备好的训练数据集的形状。
我们可以看到数据集的输入( _X_ )和输出( _Y_ )元素分别对于样本数,时间步长和变量或并行时间序列是三维的。 。
然后将每个系列的输入和输出元素并排打印,以便我们可以确认数据是按照我们的预期准备的。
```py
(5, 3, 3) (5, 2, 3)
[[10 15 25]
[20 25 45]
[30 35 65]] [[ 40 45 85]
[ 50 55 105]]
[[20 25 45]
[30 35 65]
[40 45 85]] [[ 50 55 105]
[ 60 65 125]]
[[ 30 35 65]
[ 40 45 85]
[ 50 55 105]] [[ 60 65 125]
[ 70 75 145]]
[[ 40 45 85]
[ 50 55 105]
[ 60 65 125]] [[ 70 75 145]
[ 80 85 165]]
[[ 50 55 105]
[ 60 65 125]
[ 70 75 145]] [[ 80 85 165]
[ 90 95 185]]
```
我们现在可以开发 MLP 模型来进行多变量多步预测。
除了展平输入数据的形状之外,正如我们在先前的例子中所做的那样,我们还必须平整输出数据的三维结构。这是因为 MLP 模型只能采用向量输入和输出。
```py
# flatten input
n_input = X.shape[1] * X.shape[2]
X = X.reshape((X.shape[0], n_input))
# flatten output
n_output = y.shape[1] * y.shape[2]
y = y.reshape((y.shape[0], n_output))
```
下面列出了完整的示例。
```py
# multivariate multi-step mlp example
from numpy import array
from numpy import hstack
from keras.models import Sequential
from keras.layers import Dense
# split a multivariate sequence into samples
def split_sequences(sequences, n_steps_in, n_steps_out):
X, y = list(), list()
for i in range(len(sequences)):
# find the end of this pattern
end_ix = i + n_steps_in
out_end_ix = end_ix + n_steps_out
# check if we are beyond the dataset
if out_end_ix > len(sequences):
break
# gather input and output parts of the pattern
seq_x, seq_y = sequences[i:end_ix, :], sequences[end_ix:out_end_ix, :]
X.append(seq_x)
y.append(seq_y)
return array(X), array(y)
# define input sequence
in_seq1 = array([10, 20, 30, 40, 50, 60, 70, 80, 90])
in_seq2 = array([15, 25, 35, 45, 55, 65, 75, 85, 95])
out_seq = array([in_seq1[i]+in_seq2[i] for i in range(len(in_seq1))])
# convert to [rows, columns] structure
in_seq1 = in_seq1.reshape((len(in_seq1), 1))
in_seq2 = in_seq2.reshape((len(in_seq2), 1))
out_seq = out_seq.reshape((len(out_seq), 1))
# horizontally stack columns
dataset = hstack((in_seq1, in_seq2, out_seq))
# choose a number of time steps
n_steps_in, n_steps_out = 3, 2
# convert into input/output
X, y = split_sequences(dataset, n_steps_in, n_steps_out)
# flatten input
n_input = X.shape[1] * X.shape[2]
X = X.reshape((X.shape[0], n_input))
# flatten output
n_output = y.shape[1] * y.shape[2]
y = y.reshape((y.shape[0], n_output))
# define model
model = Sequential()
model.add(Dense(100, activation='relu', input_dim=n_input))
model.add(Dense(n_output))
model.compile(optimizer='adam', loss='mse')
# fit model
model.fit(X, y, epochs=2000, verbose=0)
# demonstrate prediction
x_input = array([[60, 65, 125], [70, 75, 145], [80, 85, 165]])
x_input = x_input.reshape((1, n_input))
yhat = model.predict(x_input, verbose=0)
print(yhat)
```
运行该示例适合模型并预测超出数据集末尾的下两个时间步的三个时间步中的每一个的值。
我们希望这些系列和时间步骤的值如下:
```py
90, 95, 185
100, 105, 205
```
我们可以看到模型预测合理地接近预期值。
```py
[[ 91.28376 96.567 188.37575 100.54482 107.9219 208.108 ]
```
## 摘要
在本教程中,您了解了如何针对一系列标准时间序列预测问题开发一套多层感知器或 MLP 模型。
具体来说,你学到了:
* 如何开发单变量时间序列预测的 MLP 模型。
* 如何开发多元时间序列预测的 MLP 模型。
* 如何开发 MLP 模型进行多步时间序列预测。
你有任何问题吗?
在下面的评论中提出您的问题,我会尽力回答。
- Machine Learning Mastery 应用机器学习教程
- 5竞争机器学习的好处
- 过度拟合的简单直觉,或者为什么测试训练数据是一个坏主意
- 特征选择简介
- 应用机器学习作为一个搜索问题的温和介绍
- 为什么应用机器学习很难
- 为什么我的结果不如我想的那么好?你可能过度拟合了
- 用ROC曲线评估和比较分类器表现
- BigML评论:发现本机学习即服务平台的聪明功能
- BigML教程:开发您的第一个决策树并进行预测
- 构建生产机器学习基础设施
- 分类准确性不够:可以使用更多表现测量
- 一种预测模型的巧妙应用
- 机器学习项目中常见的陷阱
- 数据清理:将凌乱的数据转换为整洁的数据
- 机器学习中的数据泄漏
- 数据,学习和建模
- 数据管理至关重要以及为什么需要认真对待它
- 将预测模型部署到生产中
- 参数和超参数之间有什么区别?
- 测试和验证数据集之间有什么区别?
- 发现特征工程,如何设计特征以及如何获得它
- 如何开始使用Kaggle
- 超越预测
- 如何在评估机器学习算法时选择正确的测试选项
- 如何定义机器学习问题
- 如何评估机器学习算法
- 如何获得基线结果及其重要性
- 如何充分利用机器学习数据
- 如何识别数据中的异常值
- 如何提高机器学习效果
- 如何在竞争机器学习中踢屁股
- 如何知道您的机器学习模型是否具有良好的表现
- 如何布局和管理您的机器学习项目
- 如何为机器学习准备数据
- 如何减少最终机器学习模型中的方差
- 如何使用机器学习结果
- 如何解决像数据科学家这样的问题
- 通过数据预处理提高模型精度
- 处理机器学习的大数据文件的7种方法
- 建立机器学习系统的经验教训
- 如何使用机器学习清单可靠地获得准确的预测(即使您是初学者)
- 机器学习模型运行期间要做什么
- 机器学习表现改进备忘单
- 来自世界级从业者的机器学习技巧:Phil Brierley
- 模型预测精度与机器学习中的解释
- 竞争机器学习的模型选择技巧
- 机器学习需要多少训练数据?
- 如何系统地规划和运行机器学习实验
- 应用机器学习过程
- 默认情况下可重现的机器学习结果
- 10个实践应用机器学习的标准数据集
- 简单的三步法到最佳机器学习算法
- 打击机器学习数据集中不平衡类的8种策略
- 模型表现不匹配问题(以及如何处理)
- 黑箱机器学习的诱惑陷阱
- 如何培养最终的机器学习模型
- 使用探索性数据分析了解您的问题并获得更好的结果
- 什么是数据挖掘和KDD
- 为什么One-Hot在机器学习中编码数据?
- 为什么你应该在你的机器学习问题上进行抽样检查算法
- 所以,你正在研究机器学习问题......
- Machine Learning Mastery Keras 深度学习教程
- Keras 中神经网络模型的 5 步生命周期
- 在 Python 迷你课程中应用深度学习
- Keras 深度学习库的二元分类教程
- 如何用 Keras 构建多层感知器神经网络模型
- 如何在 Keras 中检查深度学习模型
- 10 个用于 Amazon Web Services 深度学习的命令行秘籍
- 机器学习卷积神经网络的速成课程
- 如何在 Python 中使用 Keras 进行深度学习的度量
- 深度学习书籍
- 深度学习课程
- 你所知道的深度学习是一种谎言
- 如何设置 Amazon AWS EC2 GPU 以训练 Keras 深度学习模型(分步)
- 神经网络中批量和迭代之间的区别是什么?
- 在 Keras 展示深度学习模型训练历史
- 基于 Keras 的深度学习模型中的dropout正则化
- 评估 Keras 中深度学习模型的表现
- 如何评价深度学习模型的技巧
- 小批量梯度下降的简要介绍以及如何配置批量大小
- 在 Keras 中获得深度学习帮助的 9 种方法
- 如何使用 Keras 在 Python 中网格搜索深度学习模型的超参数
- 用 Keras 在 Python 中使用卷积神经网络进行手写数字识别
- 如何用 Keras 进行预测
- 用 Keras 进行深度学习的图像增强
- 8 个深度学习的鼓舞人心的应用
- Python 深度学习库 Keras 简介
- Python 深度学习库 TensorFlow 简介
- Python 深度学习库 Theano 简介
- 如何使用 Keras 函数式 API 进行深度学习
- Keras 深度学习库的多类分类教程
- 多层感知器神经网络速成课程
- 基于卷积神经网络的 Keras 深度学习库中的目标识别
- 流行的深度学习库
- 用深度学习预测电影评论的情感
- Python 中的 Keras 深度学习库的回归教程
- 如何使用 Keras 获得可重现的结果
- 如何在 Linux 服务器上运行深度学习实验
- 保存并加载您的 Keras 深度学习模型
- 用 Keras 逐步开发 Python 中的第一个神经网络
- 用 Keras 理解 Python 中的有状态 LSTM 循环神经网络
- 在 Python 中使用 Keras 深度学习模型和 Scikit-Learn
- 如何使用预训练的 VGG 模型对照片中的物体进行分类
- 在 Python 和 Keras 中对深度学习模型使用学习率调度
- 如何在 Keras 中可视化深度学习神经网络模型
- 什么是深度学习?
- 何时使用 MLP,CNN 和 RNN 神经网络
- 为什么用随机权重初始化神经网络?
- Machine Learning Mastery 深度学习 NLP 教程
- 深度学习在自然语言处理中的 7 个应用
- 如何实现自然语言处理的波束搜索解码器
- 深度学习文档分类的最佳实践
- 关于自然语言处理的热门书籍
- 在 Python 中计算文本 BLEU 分数的温和介绍
- 使用编码器 - 解码器模型的用于字幕生成的注入和合并架构
- 如何用 Python 清理机器学习的文本
- 如何配置神经机器翻译的编码器 - 解码器模型
- 如何开始深度学习自然语言处理(7 天迷你课程)
- 自然语言处理的数据集
- 如何开发一种深度学习的词袋模型来预测电影评论情感
- 深度学习字幕生成模型的温和介绍
- 如何在 Keras 中定义神经机器翻译的编码器 - 解码器序列 - 序列模型
- 如何利用小实验在 Keras 中开发字幕生成模型
- 如何从头开发深度学习图片标题生成器
- 如何在 Keras 中开发基于字符的神经语言模型
- 如何开发用于情感分析的 N-gram 多通道卷积神经网络
- 如何从零开始开发神经机器翻译系统
- 如何在 Python 中用 Keras 开发基于单词的神经语言模型
- 如何开发一种预测电影评论情感的词嵌入模型
- 如何使用 Gensim 在 Python 中开发词嵌入
- 用于文本摘要的编码器 - 解码器深度学习模型
- Keras 中文本摘要的编码器 - 解码器模型
- 用于神经机器翻译的编码器 - 解码器循环神经网络模型
- 浅谈词袋模型
- 文本摘要的温和介绍
- 编码器 - 解码器循环神经网络中的注意力如何工作
- 如何利用深度学习自动生成照片的文本描述
- 如何开发一个单词级神经语言模型并用它来生成文本
- 浅谈神经机器翻译
- 什么是自然语言处理?
- 牛津自然语言处理深度学习课程
- 如何为机器翻译准备法语到英语的数据集
- 如何为情感分析准备电影评论数据
- 如何为文本摘要准备新闻文章
- 如何准备照片标题数据集以训练深度学习模型
- 如何使用 Keras 为深度学习准备文本数据
- 如何使用 scikit-learn 为机器学习准备文本数据
- 自然语言处理神经网络模型入门
- 对自然语言处理的深度学习的承诺
- 在 Python 中用 Keras 进行 LSTM 循环神经网络的序列分类
- 斯坦福自然语言处理深度学习课程评价
- 统计语言建模和神经语言模型的简要介绍
- 使用 Keras 在 Python 中进行 LSTM 循环神经网络的文本生成
- 浅谈机器学习中的转换
- 如何使用 Keras 将词嵌入层用于深度学习
- 什么是用于文本的词嵌入
- Machine Learning Mastery 深度学习时间序列教程
- 如何开发人类活动识别的一维卷积神经网络模型
- 人类活动识别的深度学习模型
- 如何评估人类活动识别的机器学习算法
- 时间序列预测的多层感知器网络探索性配置
- 比较经典和机器学习方法进行时间序列预测的结果
- 如何通过深度学习快速获得时间序列预测的结果
- 如何利用 Python 处理序列预测问题中的缺失时间步长
- 如何建立预测大气污染日的概率预测模型
- 如何开发一种熟练的机器学习时间序列预测模型
- 如何构建家庭用电自回归预测模型
- 如何开发多步空气污染时间序列预测的自回归预测模型
- 如何制定多站点多元空气污染时间序列预测的基线预测
- 如何开发时间序列预测的卷积神经网络模型
- 如何开发卷积神经网络用于多步时间序列预测
- 如何开发单变量时间序列预测的深度学习模型
- 如何开发 LSTM 模型用于家庭用电的多步时间序列预测
- 如何开发 LSTM 模型进行时间序列预测
- 如何开发多元多步空气污染时间序列预测的机器学习模型
- 如何开发多层感知器模型进行时间序列预测
- 如何开发人类活动识别时间序列分类的 RNN 模型
- 如何开始深度学习的时间序列预测(7 天迷你课程)
- 如何网格搜索深度学习模型进行时间序列预测
- 如何对单变量时间序列预测的网格搜索朴素方法
- 如何在 Python 中搜索 SARIMA 模型超参数用于时间序列预测
- 如何在 Python 中进行时间序列预测的网格搜索三次指数平滑
- 一个标准的人类活动识别问题的温和介绍
- 如何加载和探索家庭用电数据
- 如何加载,可视化和探索复杂的多变量多步时间序列预测数据集
- 如何从智能手机数据模拟人类活动
- 如何根据环境因素预测房间占用率
- 如何使用脑波预测人眼是开放还是闭合
- 如何在 Python 中扩展长短期内存网络的数据
- 如何使用 TimeseriesGenerator 进行 Keras 中的时间序列预测
- 基于机器学习算法的室内运动时间序列分类
- 用于时间序列预测的状态 LSTM 在线学习的不稳定性
- 用于罕见事件时间序列预测的 LSTM 模型体系结构
- 用于时间序列预测的 4 种通用机器学习数据变换
- Python 中长短期记忆网络的多步时间序列预测
- 家庭用电机器学习的多步时间序列预测
- Keras 中 LSTM 的多变量时间序列预测
- 如何开发和评估朴素的家庭用电量预测方法
- 如何为长短期记忆网络准备单变量时间序列数据
- 循环神经网络在时间序列预测中的应用
- 如何在 Python 中使用差异变换删除趋势和季节性
- 如何在 LSTM 中种子状态用于 Python 中的时间序列预测
- 使用 Python 进行时间序列预测的有状态和无状态 LSTM
- 长短时记忆网络在时间序列预测中的适用性
- 时间序列预测问题的分类
- Python 中长短期记忆网络的时间序列预测
- 基于 Keras 的 Python 中 LSTM 循环神经网络的时间序列预测
- Keras 中深度学习的时间序列预测
- 如何用 Keras 调整 LSTM 超参数进行时间序列预测
- 如何在时间序列预测训练期间更新 LSTM 网络
- 如何使用 LSTM 网络的 Dropout 进行时间序列预测
- 如何使用 LSTM 网络中的特征进行时间序列预测
- 如何在 LSTM 网络中使用时间序列进行时间序列预测
- 如何利用 LSTM 网络进行权重正则化进行时间序列预测
- Machine Learning Mastery 线性代数教程
- 机器学习数学符号的基础知识
- 用 NumPy 阵列轻松介绍广播
- 如何从 Python 中的 Scratch 计算主成分分析(PCA)
- 用于编码器审查的计算线性代数
- 10 机器学习中的线性代数示例
- 线性代数的温和介绍
- 用 NumPy 轻松介绍 Python 中的 N 维数组
- 机器学习向量的温和介绍
- 如何在 Python 中为机器学习索引,切片和重塑 NumPy 数组
- 机器学习的矩阵和矩阵算法简介
- 温和地介绍机器学习的特征分解,特征值和特征向量
- NumPy 对预期价值,方差和协方差的简要介绍
- 机器学习矩阵分解的温和介绍
- 用 NumPy 轻松介绍机器学习的张量
- 用于机器学习的线性代数中的矩阵类型简介
- 用于机器学习的线性代数备忘单
- 线性代数的深度学习
- 用于机器学习的线性代数(7 天迷你课程)
- 机器学习的线性代数
- 机器学习矩阵运算的温和介绍
- 线性代数评论没有废话指南
- 学习机器学习线性代数的主要资源
- 浅谈机器学习的奇异值分解
- 如何用线性代数求解线性回归
- 用于机器学习的稀疏矩阵的温和介绍
- 机器学习中向量规范的温和介绍
- 学习线性代数用于机器学习的 5 个理由
- Machine Learning Mastery LSTM 教程
- Keras中长短期记忆模型的5步生命周期
- 长短时记忆循环神经网络的注意事项
- CNN长短期记忆网络
- 逆向神经网络中的深度学习速成课程
- 可变长度输入序列的数据准备
- 如何用Keras开发用于Python序列分类的双向LSTM
- 如何开发Keras序列到序列预测的编码器 - 解码器模型
- 如何诊断LSTM模型的过度拟合和欠拟合
- 如何开发一种编码器 - 解码器模型,注重Keras中的序列到序列预测
- 编码器 - 解码器长短期存储器网络
- 神经网络中爆炸梯度的温和介绍
- 对时间反向传播的温和介绍
- 生成长短期记忆网络的温和介绍
- 专家对长短期记忆网络的简要介绍
- 在序列预测问题上充分利用LSTM
- 编辑器 - 解码器循环神经网络全局注意的温和介绍
- 如何利用长短时记忆循环神经网络处理很长的序列
- 如何在Python中对一个热编码序列数据
- 如何使用编码器 - 解码器LSTM来回显随机整数序列
- 具有注意力的编码器 - 解码器RNN体系结构的实现模式
- 学习使用编码器解码器LSTM循环神经网络添加数字
- 如何学习长短时记忆循环神经网络回声随机整数
- 具有Keras的长短期记忆循环神经网络的迷你课程
- LSTM自动编码器的温和介绍
- 如何用Keras中的长短期记忆模型进行预测
- 用Python中的长短期内存网络演示内存
- 基于循环神经网络的序列预测模型的简要介绍
- 深度学习的循环神经网络算法之旅
- 如何重塑Keras中长短期存储网络的输入数据
- 了解Keras中LSTM的返回序列和返回状态之间的差异
- RNN展开的温和介绍
- 5学习LSTM循环神经网络的简单序列预测问题的例子
- 使用序列进行预测
- 堆叠长短期内存网络
- 什么是教师强制循环神经网络?
- 如何在Python中使用TimeDistributed Layer for Long Short-Term Memory Networks
- 如何准备Keras中截断反向传播的序列预测
- 如何在使用LSTM进行训练和预测时使用不同的批量大小
- Machine Learning Mastery 机器学习算法教程
- 机器学习算法之旅
- 用于机器学习的装袋和随机森林集合算法
- 从头开始实施机器学习算法的好处
- 更好的朴素贝叶斯:从朴素贝叶斯算法中获取最多的12个技巧
- 机器学习的提升和AdaBoost
- 选择机器学习算法:Microsoft Azure的经验教训
- 机器学习的分类和回归树
- 什么是机器学习中的混淆矩阵
- 如何使用Python从头开始创建算法测试工具
- 通过创建机器学习算法的目标列表来控制
- 从头开始停止编码机器学习算法
- 在实现机器学习算法时,不要从开源代码开始
- 不要使用随机猜测作为基线分类器
- 浅谈机器学习中的概念漂移
- 温和介绍机器学习中的偏差 - 方差权衡
- 机器学习的梯度下降
- 机器学习算法如何工作(他们学习输入到输出的映射)
- 如何建立机器学习算法的直觉
- 如何实现机器学习算法
- 如何研究机器学习算法行为
- 如何学习机器学习算法
- 如何研究机器学习算法
- 如何研究机器学习算法
- 如何在Python中从头开始实现反向传播算法
- 如何用Python从头开始实现Bagging
- 如何用Python从头开始实现基线机器学习算法
- 如何在Python中从头开始实现决策树算法
- 如何用Python从头开始实现学习向量量化
- 如何利用Python从头开始随机梯度下降实现线性回归
- 如何利用Python从头开始随机梯度下降实现Logistic回归
- 如何用Python从头开始实现机器学习算法表现指标
- 如何在Python中从头开始实现感知器算法
- 如何在Python中从零开始实现随机森林
- 如何在Python中从头开始实现重采样方法
- 如何用Python从头开始实现简单线性回归
- 如何用Python从头开始实现堆栈泛化(Stacking)
- K-Nearest Neighbors for Machine Learning
- 学习机器学习的向量量化
- 机器学习的线性判别分析
- 机器学习的线性回归
- 使用梯度下降进行机器学习的线性回归教程
- 如何在Python中从头开始加载机器学习数据
- 机器学习的Logistic回归
- 机器学习的Logistic回归教程
- 机器学习算法迷你课程
- 如何在Python中从头开始实现朴素贝叶斯
- 朴素贝叶斯机器学习
- 朴素贝叶斯机器学习教程
- 机器学习算法的过拟合和欠拟合
- 参数化和非参数机器学习算法
- 理解任何机器学习算法的6个问题
- 在机器学习中拥抱随机性
- 如何使用Python从头开始扩展机器学习数据
- 机器学习的简单线性回归教程
- 有监督和无监督的机器学习算法
- 用于机器学习的支持向量机
- 在没有数学背景的情况下理解机器学习算法的5种技术
- 最好的机器学习算法
- 教程从头开始在Python中实现k-Nearest Neighbors
- 通过从零开始实现它们来理解机器学习算法(以及绕过坏代码的策略)
- 使用随机森林:在121个数据集上测试179个分类器
- 为什么从零开始实现机器学习算法
- Machine Learning Mastery 机器学习入门教程
- 机器学习入门的四个步骤:初学者入门与实践的自上而下策略
- 你应该培养的 5 个机器学习领域
- 一种选择机器学习算法的数据驱动方法
- 机器学习中的分析与数值解
- 应用机器学习是一种精英政治
- 机器学习的基本概念
- 如何成为数据科学家
- 初学者如何在机器学习中弄错
- 机器学习的最佳编程语言
- 构建机器学习组合
- 机器学习中分类与回归的区别
- 评估自己作为数据科学家并利用结果建立惊人的数据科学团队
- 探索 Kaggle 大师的方法论和心态:对 Diogo Ferreira 的采访
- 扩展机器学习工具并展示掌握
- 通过寻找地标开始机器学习
- 温和地介绍预测建模
- 通过提供结果在机器学习中获得梦想的工作
- 如何开始机器学习:自学蓝图
- 开始并在机器学习方面取得进展
- 应用机器学习的 Hello World
- 初学者如何使用小型项目开始机器学习并在 Kaggle 上进行竞争
- 我如何开始机器学习? (简短版)
- 我是如何开始机器学习的
- 如何在机器学习中取得更好的成绩
- 如何从在银行工作到担任 Target 的高级数据科学家
- 如何学习任何机器学习工具
- 使用小型目标项目深入了解机器学习工具
- 获得付费申请机器学习
- 映射机器学习工具的景观
- 机器学习开发环境
- 机器学习金钱
- 程序员的机器学习
- 机器学习很有意思
- 机器学习是 Kaggle 比赛
- 机器学习现在很受欢迎
- 机器学习掌握方法
- 机器学习很重要
- 机器学习 Q& A:概念漂移,更好的结果和学习更快
- 缺乏自学机器学习的路线图
- 机器学习很重要
- 快速了解任何机器学习工具(即使您是初学者)
- 机器学习工具
- 找到你的机器学习部落
- 机器学习在一年
- 通过竞争一致的大师 Kaggle
- 5 程序员在机器学习中开始犯错误
- 哲学毕业生到机器学习从业者(Brian Thomas 采访)
- 机器学习入门的实用建议
- 实用机器学习问题
- 使用来自 UCI 机器学习库的数据集练习机器学习
- 使用秘籍的任何机器学习工具快速启动
- 程序员可以进入机器学习
- 程序员应该进入机器学习
- 项目焦点:Shashank Singh 的人脸识别
- 项目焦点:使用 Mahout 和 Konstantin Slisenko 进行堆栈交换群集
- 机器学习自学指南
- 4 个自学机器学习项目
- ÁlvaroLemos 如何在数据科学团队中获得机器学习实习
- 如何思考机器学习
- 现实世界机器学习问题之旅
- 有关机器学习的有用知识
- 如果我没有学位怎么办?
- 如果我不是一个优秀的程序员怎么办?
- 如果我不擅长数学怎么办?
- 为什么机器学习算法会处理以前从未见过的数据?
- 是什么阻碍了你的机器学习目标?
- 什么是机器学习?
- 机器学习适合哪里?
- 为什么要进入机器学习?
- 研究对您来说很重要的机器学习问题
- 你这样做是错的。为什么机器学习不必如此困难
- Machine Learning Mastery Sklearn 教程
- Scikit-Learn 的温和介绍:Python 机器学习库
- 使用 Python 管道和 scikit-learn 自动化机器学习工作流程
- 如何以及何时使用带有 scikit-learn 的校准分类模型
- 如何比较 Python 中的机器学习算法与 scikit-learn
- 用于机器学习开发人员的 Python 崩溃课程
- 用 scikit-learn 在 Python 中集成机器学习算法
- 使用重采样评估 Python 中机器学习算法的表现
- 使用 Scikit-Learn 在 Python 中进行特征选择
- Python 中机器学习的特征选择
- 如何使用 scikit-learn 在 Python 中生成测试数据集
- scikit-learn 中的机器学习算法秘籍
- 如何使用 Python 处理丢失的数据
- 如何开始使用 Python 进行机器学习
- 如何使用 Scikit-Learn 在 Python 中加载数据
- Python 中概率评分方法的简要介绍
- 如何用 Scikit-Learn 调整算法参数
- 如何在 Mac OS X 上安装 Python 3 环境以进行机器学习和深度学习
- 使用 scikit-learn 进行机器学习简介
- 从 shell 到一本带有 Fernando Perez 单一工具的书的 IPython
- 如何使用 Python 3 为机器学习开发创建 Linux 虚拟机
- 如何在 Python 中加载机器学习数据
- 您在 Python 中的第一个机器学习项目循序渐进
- 如何使用 scikit-learn 进行预测
- 用于评估 Python 中机器学习算法的度量标准
- 使用 Pandas 为 Python 中的机器学习准备数据
- 如何使用 Scikit-Learn 为 Python 机器学习准备数据
- 项目焦点:使用 Artem Yankov 在 Python 中进行事件推荐
- 用于机器学习的 Python 生态系统
- Python 是应用机器学习的成长平台
- Python 机器学习书籍
- Python 机器学习迷你课程
- 使用 Pandas 快速和肮脏的数据分析
- 使用 Scikit-Learn 重新调整 Python 中的机器学习数据
- 如何以及何时使用 ROC 曲线和精确调用曲线进行 Python 分类
- 使用 scikit-learn 在 Python 中保存和加载机器学习模型
- scikit-learn Cookbook 书评
- 如何使用 Anaconda 为机器学习和深度学习设置 Python 环境
- 使用 scikit-learn 在 Python 中进行 Spot-Check 分类机器学习算法
- 如何在 Python 中开发可重复使用的抽样检查算法框架
- 使用 scikit-learn 在 Python 中进行 Spot-Check 回归机器学习算法
- 使用 Python 中的描述性统计来了解您的机器学习数据
- 使用 OpenCV,Python 和模板匹配来播放“哪里是 Waldo?”
- 使用 Pandas 在 Python 中可视化机器学习数据
- Machine Learning Mastery 统计学教程
- 浅谈计算正态汇总统计量
- 非参数统计的温和介绍
- Python中常态测试的温和介绍
- 浅谈Bootstrap方法
- 浅谈机器学习的中心极限定理
- 浅谈机器学习中的大数定律
- 机器学习的所有统计数据
- 如何计算Python中机器学习结果的Bootstrap置信区间
- 浅谈机器学习的Chi-Squared测试
- 机器学习的置信区间
- 随机化在机器学习中解决混杂变量的作用
- 机器学习中的受控实验
- 机器学习统计学速成班
- 统计假设检验的关键值以及如何在Python中计算它们
- 如何在机器学习中谈论数据(统计学和计算机科学术语)
- Python中数据可视化方法的简要介绍
- Python中效果大小度量的温和介绍
- 估计随机机器学习算法的实验重复次数
- 机器学习评估统计的温和介绍
- 如何计算Python中的非参数秩相关性
- 如何在Python中计算数据的5位数摘要
- 如何在Python中从头开始编写学生t检验
- 如何在Python中生成随机数
- 如何转换数据以更好地拟合正态分布
- 如何使用相关来理解变量之间的关系
- 如何使用统计信息识别数据中的异常值
- 用于Python机器学习的随机数生成器简介
- k-fold交叉验证的温和介绍
- 如何计算McNemar的比较两种机器学习量词的测试
- Python中非参数统计显着性测试简介
- 如何在Python中使用参数统计显着性测试
- 机器学习的预测间隔
- 应用统计学与机器学习的密切关系
- 如何使用置信区间报告分类器表现
- 统计数据分布的简要介绍
- 15 Python中的统计假设检验(备忘单)
- 统计假设检验的温和介绍
- 10如何在机器学习项目中使用统计方法的示例
- Python中统计功效和功耗分析的简要介绍
- 统计抽样和重新抽样的简要介绍
- 比较机器学习算法的统计显着性检验
- 机器学习中统计容差区间的温和介绍
- 机器学习统计书籍
- 评估机器学习模型的统计数据
- 机器学习统计(7天迷你课程)
- 用于机器学习的简明英语统计
- 如何使用统计显着性检验来解释机器学习结果
- 什么是统计(为什么它在机器学习中很重要)?
- Machine Learning Mastery 时间序列入门教程
- 如何在 Python 中为时间序列预测创建 ARIMA 模型
- 用 Python 进行时间序列预测的自回归模型
- 如何回溯机器学习模型的时间序列预测
- Python 中基于时间序列数据的基本特征工程
- R 的时间序列预测热门书籍
- 10 挑战机器学习时间序列预测问题
- 如何将时间序列转换为 Python 中的监督学习问题
- 如何将时间序列数据分解为趋势和季节性
- 如何用 ARCH 和 GARCH 模拟波动率进行时间序列预测
- 如何将时间序列数据集与 Python 区分开来
- Python 中时间序列预测的指数平滑的温和介绍
- 用 Python 进行时间序列预测的特征选择
- 浅谈自相关和部分自相关
- 时间序列预测的 Box-Jenkins 方法简介
- 用 Python 简要介绍时间序列的时间序列预测
- 如何使用 Python 网格搜索 ARIMA 模型超参数
- 如何在 Python 中加载和探索时间序列数据
- 如何使用 Python 对 ARIMA 模型进行手动预测
- 如何用 Python 进行时间序列预测的预测
- 如何使用 Python 中的 ARIMA 进行样本外预测
- 如何利用 Python 模拟残差错误来纠正时间序列预测
- 使用 Python 进行数据准备,特征工程和时间序列预测的移动平均平滑
- 多步时间序列预测的 4 种策略
- 如何在 Python 中规范化和标准化时间序列数据
- 如何利用 Python 进行时间序列预测的基线预测
- 如何使用 Python 对时间序列预测数据进行功率变换
- 用于时间序列预测的 Python 环境
- 如何重构时间序列预测问题
- 如何使用 Python 重新采样和插值您的时间序列数据
- 用 Python 编写 SARIMA 时间序列预测
- 如何在 Python 中保存 ARIMA 时间序列预测模型
- 使用 Python 进行季节性持久性预测
- 基于 ARIMA 的 Python 历史规模敏感性预测技巧分析
- 简单的时间序列预测模型进行测试,这样你就不会欺骗自己
- 标准多变量,多步骤和多站点时间序列预测问题
- 如何使用 Python 检查时间序列数据是否是固定的
- 使用 Python 进行时间序列数据可视化
- 7 个机器学习的时间序列数据集
- 时间序列预测案例研究与 Python:波士顿每月武装抢劫案
- Python 的时间序列预测案例研究:巴尔的摩的年度用水量
- 使用 Python 进行时间序列预测研究:法国香槟的月销售额
- 使用 Python 的置信区间理解时间序列预测不确定性
- 11 Python 中的经典时间序列预测方法(备忘单)
- 使用 Python 进行时间序列预测表现测量
- 使用 Python 7 天迷你课程进行时间序列预测
- 时间序列预测作为监督学习
- 什么是时间序列预测?
- 如何使用 Python 识别和删除时间序列数据的季节性
- 如何在 Python 中使用和删除时间序列数据中的趋势信息
- 如何在 Python 中调整 ARIMA 参数
- 如何用 Python 可视化时间序列残差预测错误
- 白噪声时间序列与 Python
- 如何通过时间序列预测项目
- Machine Learning Mastery XGBoost 教程
- 通过在 Python 中使用 XGBoost 提前停止来避免过度拟合
- 如何在 Python 中调优 XGBoost 的多线程支持
- 如何配置梯度提升算法
- 在 Python 中使用 XGBoost 进行梯度提升的数据准备
- 如何使用 scikit-learn 在 Python 中开发您的第一个 XGBoost 模型
- 如何在 Python 中使用 XGBoost 评估梯度提升模型
- 在 Python 中使用 XGBoost 的特征重要性和特征选择
- 浅谈机器学习的梯度提升算法
- 应用机器学习的 XGBoost 简介
- 如何在 macOS 上为 Python 安装 XGBoost
- 如何在 Python 中使用 XGBoost 保存梯度提升模型
- 从梯度提升开始,比较 165 个数据集上的 13 种算法
- 在 Python 中使用 XGBoost 和 scikit-learn 进行随机梯度提升
- 如何使用 Amazon Web Services 在云中训练 XGBoost 模型
- 在 Python 中使用 XGBoost 调整梯度提升的学习率
- 如何在 Python 中使用 XGBoost 调整决策树的数量和大小
- 如何在 Python 中使用 XGBoost 可视化梯度提升决策树
- 在 Python 中开始使用 XGBoost 的 7 步迷你课程